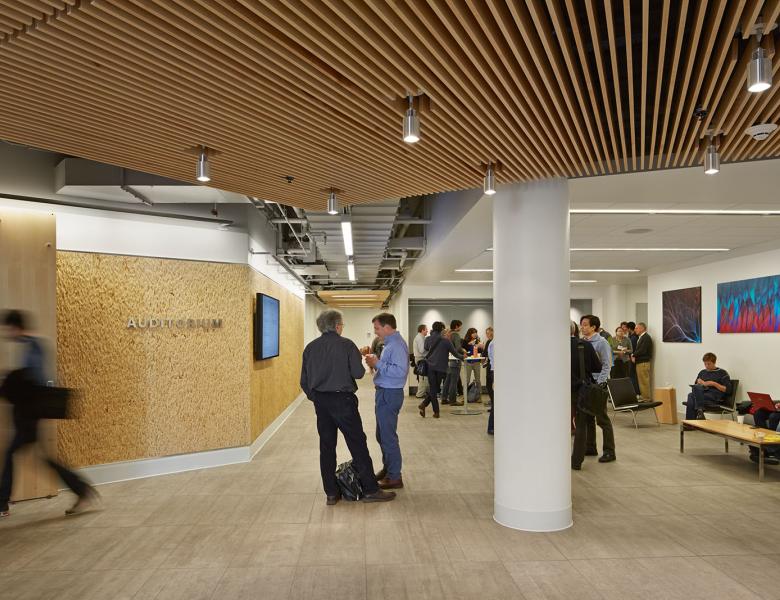
Abstract
In the contemporary realm of machine learning, applications such as adversarial attacks and multi-agent reinforcement learning can be viewed as intricate multi-agent games, where Nash equilibria represent optimal system states. Amidst the evident non-convex loss landscape of these games, a latent convex structure emerges, offering a potential route to convergence. In this talk, we will introduce a pioneering first-order method adept at leveraging this concealed structure, ensuring convergence to a Nash equilibrium in such non-convex games.
Merging traditional game theory with neural network dynamism, our work addresses the obfuscated nature of AI architectures by navigating the maze of control and latent variables, charting a path for convergence in hidden monotone games.