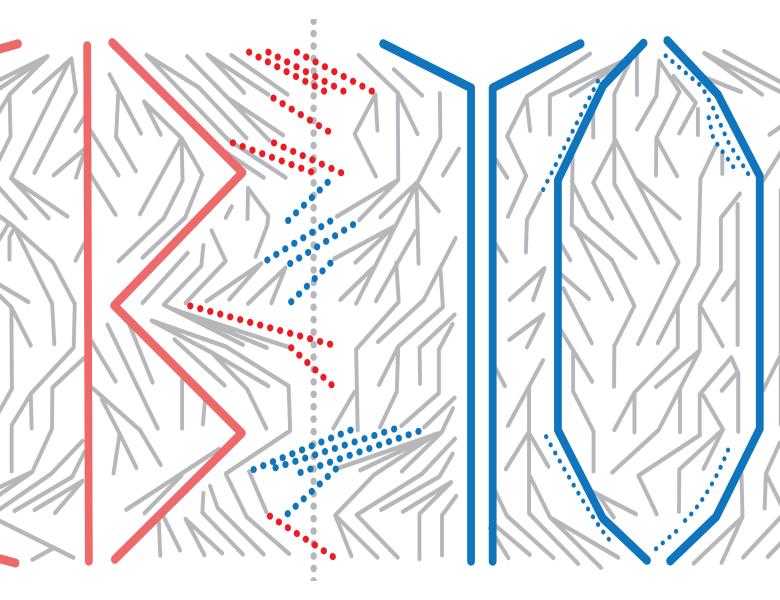
Abstract
Variation in human DNA sequences account for a significant amount of genetic risk factors for common disease such as hypertension, diabetes, Alzheimer's disease, and cancer. Identifying the human sequence variation that makes up the genetic basis of common disease will have a tremendous impact on medicine in many ways. Recent efforts to identify these genetic factors through large scale association studies which compare information on variation between a set of healthy and diseased individuals have been remarkably successful. However, despite the success of these initial studies, many challenges and open questions remain on how to design and analyze the results of association studies. As several recent studies have demonstrated, confounding factors such as population structure and measurement errors can complicate genetics analysis by causing many spurious associations. Yet little is understood about how these confounding factors affect analyses and how to correct for these factors. In this talk I will discuss several recently developed methods based on linear mixed models for correcting for both known and unknown confounding factors in genetic studies.