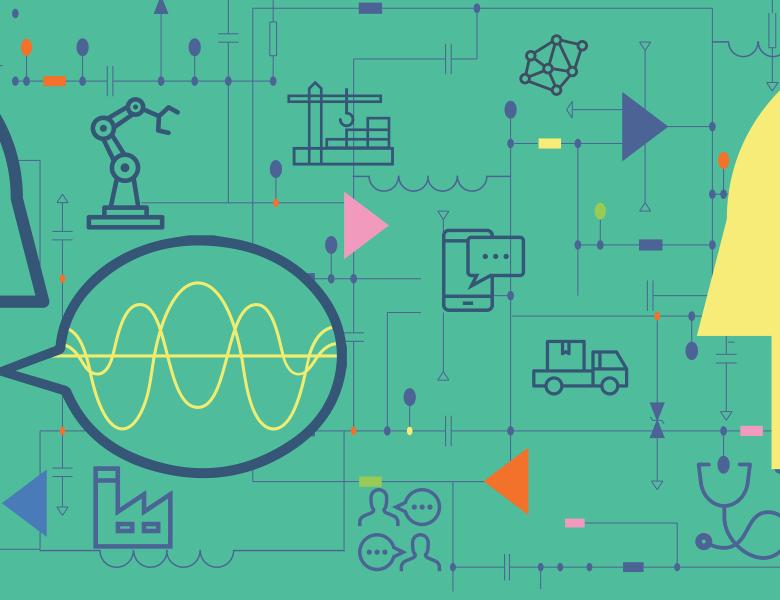
Abstract
Temporal difference methods are the workhorses of policy evaluation with linear function approximation, and it is well-known that stochastic approximation procedures of this form converge to the solution of a projected fixed point equation. Classical work by Tsitsiklis and Van Roy showed that this solution can in general suffer error that is significantly larger—by a horizon-dependent multiplicative factor—than the minimum attainable approximation error. Similar bounds can be traced even further back in the numerical analysis literature on Galerkin methods.  In the parlance of statistical machine learning, these results constitute nonstandard oracle inequalities, in which the approximation factor is much larger than a constant. More importantly, the eventual performance of these algorithms is often governed by their approximation error, begging the question of whether alternative methods can perform better along this axis. This talk will present an information-theoretic investigation into this question for general projected linear equations, and complement this with instance-dependent upper and lower bounds on both the approximation and estimation errors for a family of stochastic approximation methods. Consequences for policy evaluation will also be discussed. Joint work with Wenlong Mou and Martin Wainwright, based on the following preprint: https://arxiv.org/abs/2012.05299