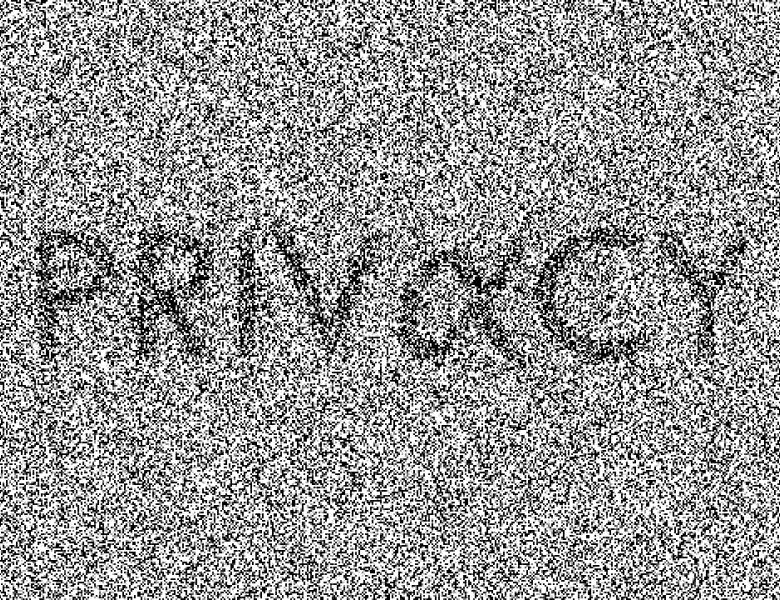
Abstract
The adoption of differential privacy in the Census operations has been controversial. While the ongoing academic debate surrounding the trade-offs raised by differential privacy is vital, the focus of much of the analysis on errors that differential privacy may cause in various applications of census statistics misses an important nuance. Census counts have always been noisy: estimates from the ACS, Decennial Census, and other data products used for critical policy decisions contain sampling, measurement, and other errors. Through a case study on the Title I education funding allocation, we evaluate the impact of differential privacy in context by empirically contrasting deviations in the outcomes of policy brought on by differential privacy (privacy deviations) to those resulting from an underlying error in estimation (data deviations). Our results demonstrate how privacy-motivated noise injection is only a marginal increase to already-existing uncertainty in data --- and that the problem of differential privacy may expose flaws in the ways our political and government infrastructures handle uncertain inputs, to the detriment of marginalized communities. This is joint work with Ryan Steed, Terrance Liu, and Alessandro Acquisti.