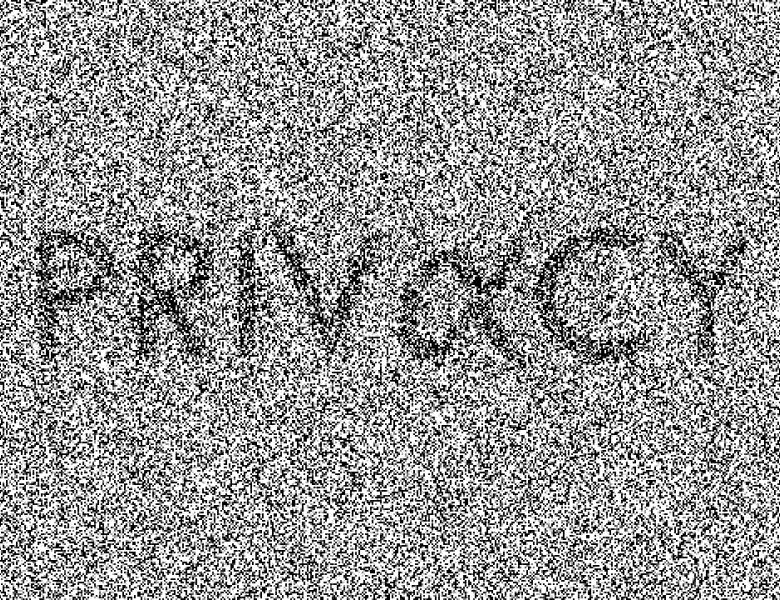
Abstract
While the concept of differential privacy generated a lot of interest not only among computer scientists, but also in the statistical community, actual applications of differential privacy at statistical agencies have been sparse until recently. This changed, when the U.S. Census Bureau announced that some of the flagship products of the Bureau (most notably the 2020 Census) will be protected using mechanisms fulfilling the requirements of differential privacy.
A key challenge which needs to be addressed when looking at the problem from a statistical perspective is how to obtain statistically valid inferences from the protected data, i.e. how to take the extra uncertainty from the protection mechanism into account. In this talk I will present some ideas to address this problem. The methodology will be illustrated using administrative data gathered by the German Federal Employment Agency. Detailed geocoding information has been added to this database recently and plans call for making this valuable source of information available to the scientific community. I will discuss which steps are required to generate privacy protected microdata for this database and illustrate, which problems arise in practical settings when following the recommendations in the literature for generating differentially private microdata. I will propose some strategies to overcome these limitations and show how valid inferences for means and totals can be obtained from the protected dataset.