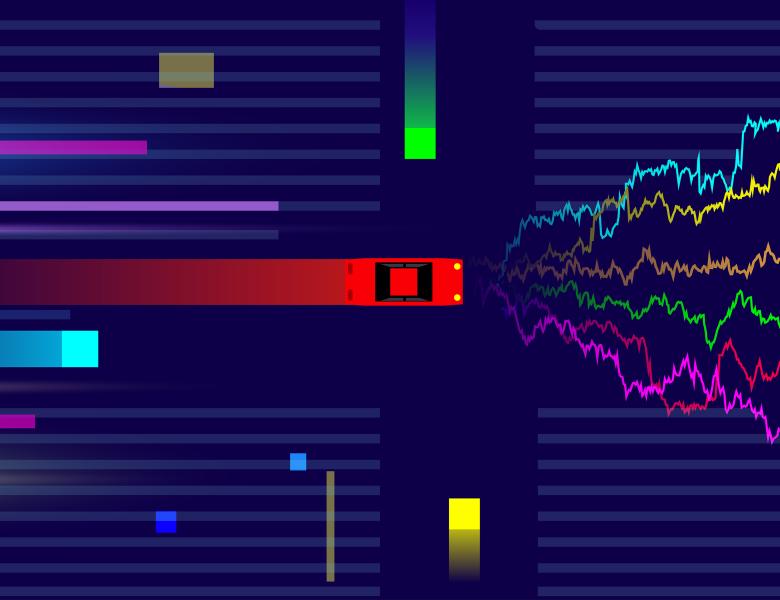
Abstract
Almost all work in reinforcement learning focuses on one of two settings: the online case, where an algorithm can gather data and near immediately update its policy, and the offline case, where an algorithm must learn exclusively from existing historical data. Yet many applied areas, including education, healthcare and developmental economics, have a history of running experiments to assess the impact of different potential interventions, for the purpose of selecting decision policies for future wider-scale deployment. We can view this as the batch or limited deployment setting, where one or more explicit experiments may be conducted, in order to gather information to identify near optimal policies. Towards progress for these settings, we introduce the first, to our knowledge, algorithm for doing this for linear contextual multi-armed bandits where the aim is to minimize the sample complexity needed to learn a near-optimal policy. We provide theoretical bounds and encouraging empirical results, even when the domain violates the linearity assumptions. This is joint work with Andrea Zanette, Kefan Dong, Jonathan Lee and Matt Joerke.