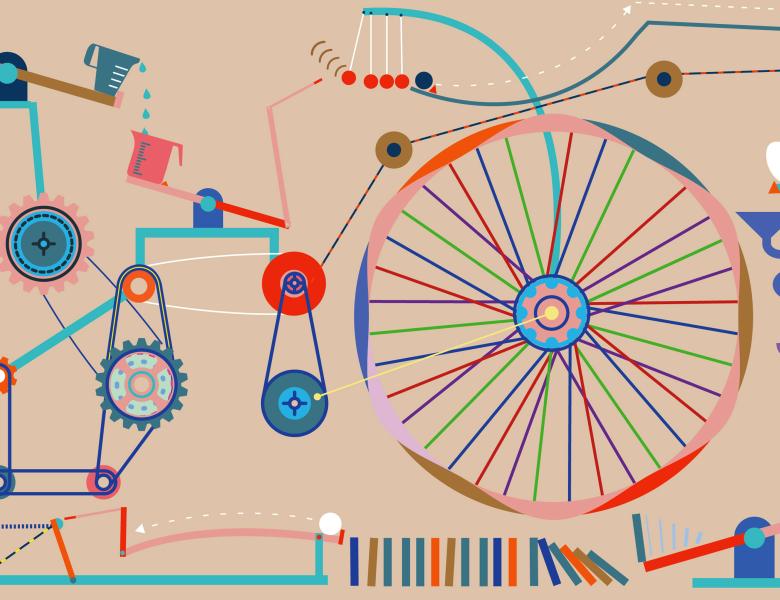
Abstract
Policymakers and experimental researchers may face the problem of assessing multiple competing treatment policies, where ex ante they have limited information about the distribution of response under the different policies, but ex post they are most interested in the policy that will turn out to be the most effective. Adaptive experimental designs allow researchers to more quickly identify the best policy, to reduce assignment to ineffective policies, and to collect the most data under the most effective policies. In addition to these objectives, policymakers and researchers may also be interested in inferential targets such as causal effects with respect to a control condition, constructing valid confidence intervals, and testing hypotheses with respect to learned policies. There is a growing literature that uses statistical tools such as re-weighting to construct relevant estimates from adaptively collected data. However, for adaptive designs to best serve inferential objectives, most adaptive algorithms should not be used out of the box, and researchers must also carefully consider design parameters. Unlike in conventional randomized control trials, researchers cannot conduct power calculations with full knowledge of how treatment will be allocated. When comparison to a control condition is of interest, researchers must also consider control assignment. Structured simulations and careful design documents can ensure that experiments using adaptive designs will appropriately serve the inferential objectives of policymakers and researchers.