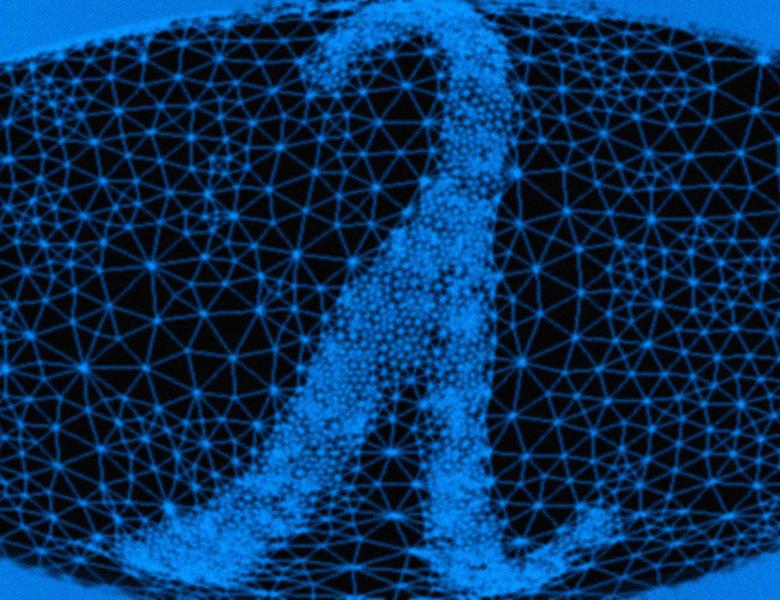
Abstract
We study a variety of spectral-based information diffusion problems that occur in the literature as relaxations of min-cut objectives. This allows us to describe two methods to make these diffusion-based algorithms more robust to the details of graph constructions, errors in node labeling, degree variability, and a variety of other real-world heterogeneities. The two methods are solidly grounded in the theory of spectral graph methods and include a rank-based label assignment strategy and a scalable-approximation algorithm for the basic diffusion algorithm that implicitly has sparsity-based regularization properties. In addition to describing the framework, we provide an empirical study these methods on a digit prediction problem as well as a variety of other pedagogical examples.