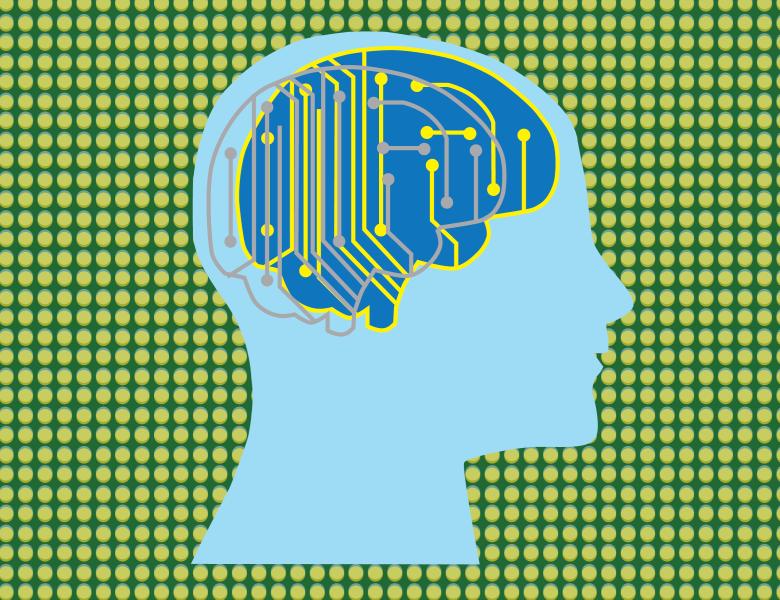
Abstract
The ability to predict future states of the world is essential for planning behavior, and it is arguably a central pillar of intelligence. In the field of sensory neuroscience, "predictive coding" -- the notion that circuits in cerebral actively predict their own activity -- has been an influential theoretical framework for understanding visual cortex. In my talk, I will bring together the idea of predictive coding with modern tools of machine learning to build practical, working vision models that predict their inputs in both space and time. These networks learn to predict future frames in a video sequence, with each layer in the network making local predictions and only forwarding deviations from those predictions to subsequent network layers. We show that these networks are able to robustly learn to predict the movement of synthetic (rendered) objects, and that in doing so, the networks learn internal representations that are useful for decoding latent object parameters (e.g. pose) that support object recognition with fewer training views. We also show that these networks can scale to complex natural image streams (car-mounted camera videos), capturing key aspects of both egocentric movement and the movement of objects in the visual scene, and generalizing well across video datasets. These results suggest that prediction represents a powerful framework for unsupervised learning, allowing for implicit learning of object and scene structure. At the same time, we find that models trained for prediction also recapitulate a wide variety of findings in neuroscience and psychology, providing a touch point between deep learning and empirical neuroscience data.
Session Chair: Christos Papadimitriou