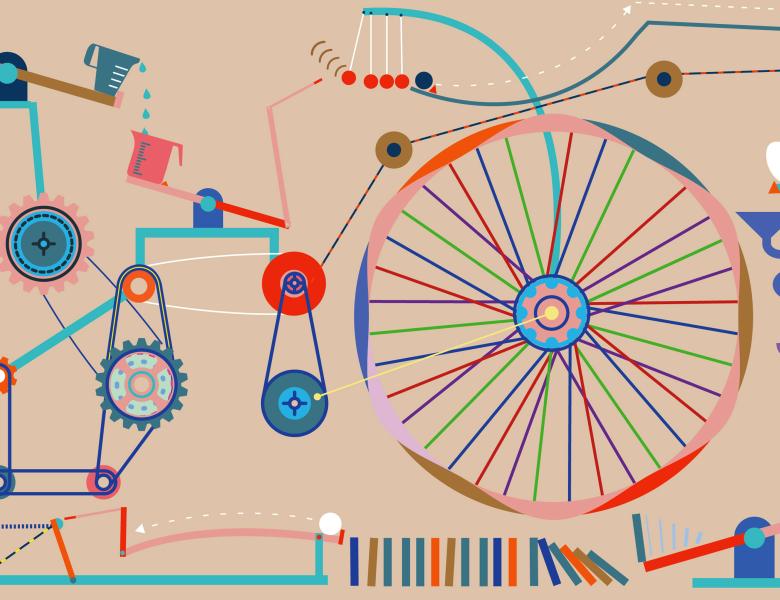
Abstract
We provide a novel characterization of estimators that combine balancing weights, which estimate inverse propensity score weights directly, and outcome modeling; examples include automatic debiased machine learning and augmented balancing weights. When the outcome and weighting models are both linear in some (possibly infinite) basis, the augmented estimator is equivalent to a single linear model with coefficients that combine the original outcome model coefficients and OLS; in many settings, the augmented estimator collapses to OLS alone. When the weighting model is kernel ridge regression, we show that the augmented estimator is always a form of overfitting. And when both outcome and weighting models are kernel ridge, the combined estimator is equivalent to a single, undersmoothed kernel ridge regression; this holds both numerically and statistically. When the weighting model is lasso regression, we give closed-form expressions for special cases and demonstrate a ``double selection'' property. Finally, we extend these results to general estimands via the Riesz representer. Our framework ``opens the black box'' on these increasingly popular estimators and provides important insights into estimation choices for augmented balancing weights.