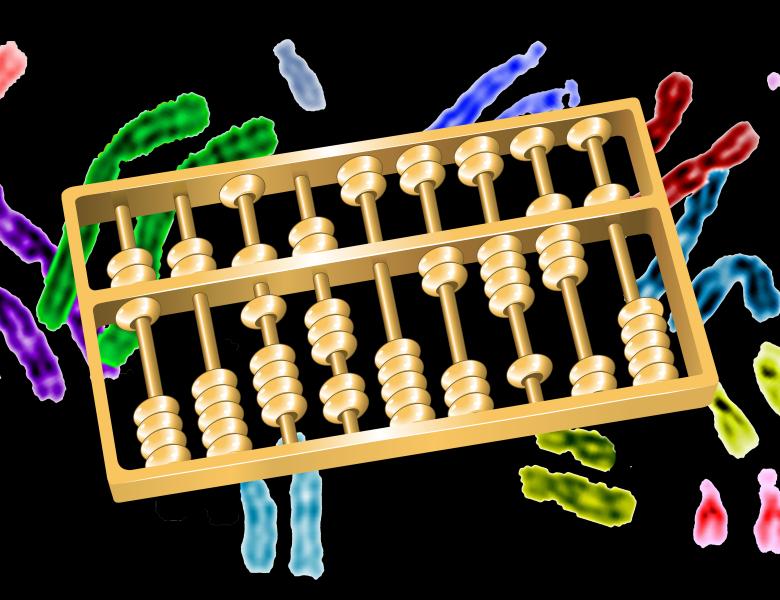
Abstract
In January of this year, the number of publicly available gene expression assays topped 1.9 million. Near the time of this workshop, there will be 2 million samples available. Our lab is developing algorithms to integrate these data into models of the underlying biological systems that can be used to discover the pathways and processes that play roles in cells' responses to their environment. One of the methods that we've developed, ADAGE, adapts techniques from deep learning to perform unsupervised extraction of co-regulated modules from noisy publicly available data. Once trained, the ADAGE model can be applied to newly generated data to reveal the pathways altered by a newly performed experiment. This analysis, the output of which resembles a pathway analysis from commonly used software, is unsupervised and entirely data-driven. This means that the technique can be applied to systems for which gene expression data exist but no curated knowledge bases are available. Subsampling analysis suggests that there are currently about 150 organisms for which enough data exists to construct an ADAGE model, and for many of these curated knowledge bases are unavailable or limited to homology-transferred annotations. In addition to continuing methodological developments, we are also developing the software infrastructure to provide data-driven pathway analysis for this set of organisms.