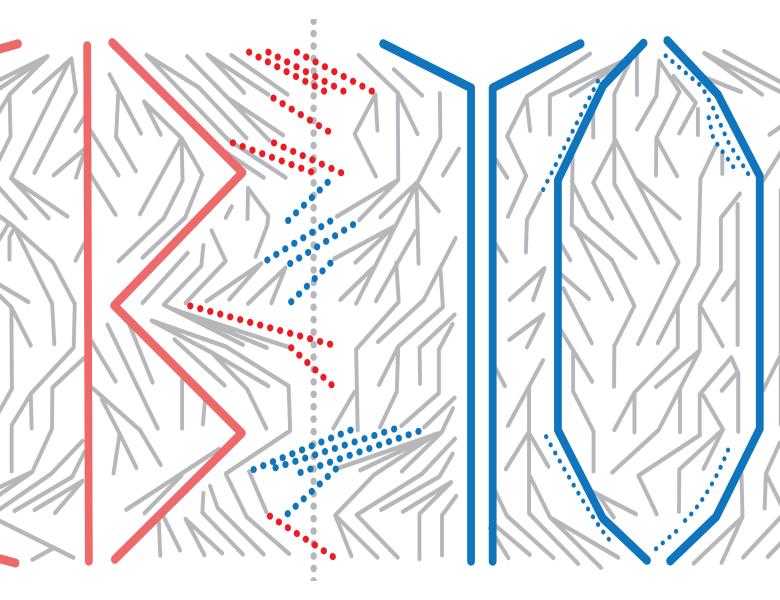
Abstract
Current evolutionary theory describes a Darwinian machine – i.e., heritable variation in reproductive success that assumes fixed mechanisms of variation and selection operating on a fixed reproductive unit. But, in fact, none of these mechanisms is fixed in nature. For example, the distribution of phenotypic variation changes over evolutionary time as a result of the evolution of development, the selective pressures on traits change as a result of the evolution of ecological interactions, and even the identity of the evolutionary unit changes as a result of the evolution of new reproductive strategies and new mechanisms of inheritance. The circular causality implied by an evolutionary process that alters its own mechanisms results in conceptual difficulties and controversies in many areas of evolutionary biology. However, in computer science, the idea that an algorithmic process can improve over time as a function of past experience, including its own past behaviour, has been thoroughly studied in the field of machine learning. Although many techniques in machine learning may seem unbiological at first, many of them are based on incrementally improving the fit of a model to data. Since incremental improvement is something natural selection can do, it only remains to be shown that evolution has access to a suitable model space.
Our work has shown that the selective pressures acting on regulatory interactions in a gene network are equivalent to (supervised) associative learning (Hebbian learning) well-studied in the context of neural network research (1). Accordingly a gene network can evolve a distributed associative memory of past selective environments and the principles of associative generalisation then help us understand how this internalised model of the past can modify the distribution of phenotypic variation in a manner that makes subsequent evolution faster and better (2,3). We can then use textbook machine learning results to understand the affordances and limitations of these phenomena including the tendency of evolution to 'over-fit' to past selective environments, failing to generalise to novel future environments, and the conditions that alleviate this and hence improve evolvability.
We have also shown that the selective pressures acting on ecological interactions in an ecosystem are equivalent to (unsupervised) associative learning, meaning that ecosystems can (also) form attractors that represent a generalised memory of past ecological states/environmental conditions (4). This shows that the organisation of ecological networks is not necessarily arbitrary but can be conditioned by past experience in a systematic manner. Beyond this, the fact that unsupervised learning can be used to 'prime' good performance at supervised learning tasks then suggests a systematic mechanism by which evolution before a transition in individuality can facilitate evolution after a transition in individuality (5,6). That is, individual selection in a system that is not yet an evolutionary unit can systematically prime for effective higher-level selection. We show that these principles can solve combinatorial optimisation problems that cannot be solved by any one level of selection (6,7).
- Watson, R. A., Wagner, G. P., Pavlicev, M., Weinreich, D. M., & Mills, R. (2014). The Evolution of Phenotypic Correlations and “Developmental Memory”. Evolution. (to appear)
- Watson, R. A., Buckley, C. L., & Mills, R. (2011). Optimization in “self‐modeling” complex adaptive systems. Complexity, 16(5), 17-26.
- Watson, R. A., Mills, R., & Buckley, C. L. (2011). Global adaptation in networks of selfish components: Emergent associative memory at the system scale. Artificial Life, 17(3), 147-166.
- Watson, R.A., Power, D.A., Szathmáry, E., Mills, R., Powers, S.T., Czapp, B. (in prep) The evolution of ecological relationships and principles of distributed learning in ecosystems
- Watson, R.A., R. Mills, R., C.L. Buckley, K. Kouvaris, A. Jackson, S.T. Powers, C. Cox, S. Tudge, P. Ryan, A. Davies (2014b) Evolutionary Connectionism: How the principles of associative learning operate in the evolution of developmental interactions, the evolution of ecological relationships and the evolution of new levels of individuality. Technical Report, ECS, University of Southampton, Southampton, U.K.
- Watson, R. A., Palmius, N., Mills, R., Powers, S. T., & Penn, A. (2011). Can selfish symbioses effect higher-level selection?. In Advances in Artificial Life. Darwin Meets von Neumann (pp. 27-36). Springer Berlin Heidelberg.
- Watson, R. A., Mills, R., & Buckley, C. L. (2011). Transformations in the scale of behavior and the global optimization of constraints in adaptive networks. Adaptive Behavior, 19(4), 227-249.
Joint work with Eors Szathmary, Daniel Power, Gunter Wagner, Kostas Kouvaris, Louis Kounios, Rob Mills, Chris Cox, and Adam Jackson.