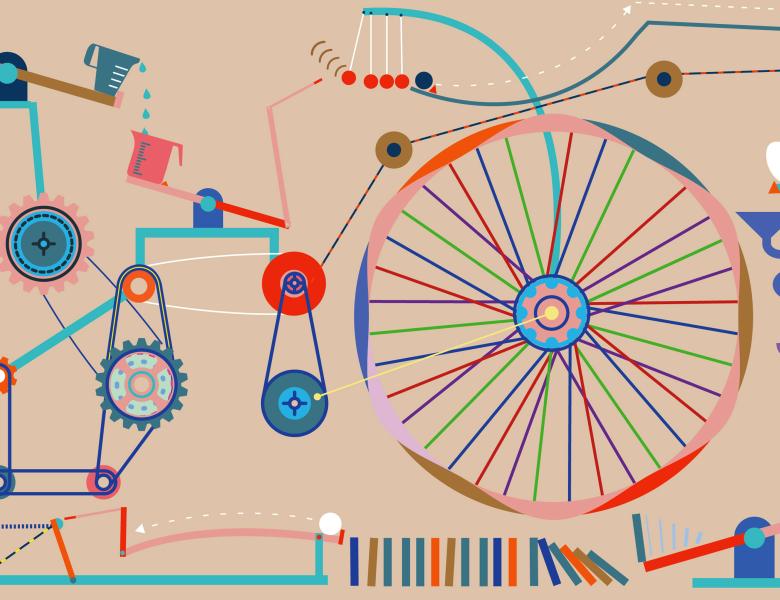
Abstract
Causal graphical models are used many scientific domains to represent important causal assumptions about the processes that underlie collected data. The focus of this work is on graphical structure learning (a.k.a. causal discovery or model selection) for the “downstream” purpose of using the estimated graph for a subsequent causal inference tasks, such as establishing the identifying formula for some causal effect of interest and then estimating it. An obstacle to having confidence in existing procedures in applied health science settings is that they have a tendency to estimate structures that are overly sparse, i.e., missing too many edges. However, statistical “caution” (or “conservativism”) would err on the side of more dense graphs rather than more sparse graphs. This paper proposes to reformulate the conditional independence hypothesis tests of classical constraint-based algorithms as equivalence tests: test the null hypothesis of association greater than some (user-chosen, sample-size dependent) threshold, rather than test the null of no association. We argue this addresses several important statistical issues in applied causal model selection and leads to procedures with desirable behaviors and properties.