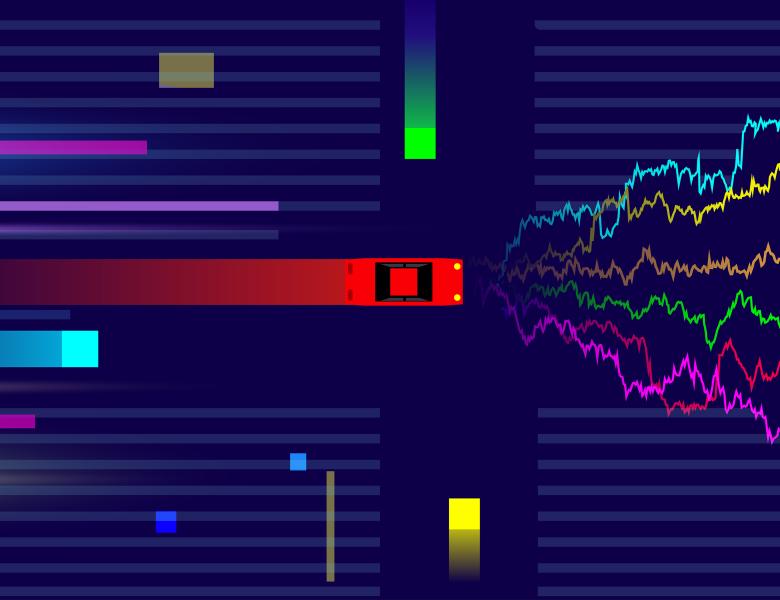
Abstract
Recent research proposed algorithms that assign refugees and asylum seekers to geographic locations in a manner that maximizes the global average employment. While these algorithms can have substantial overall positive impact, using data from industry collaborators we show that the impact of them can vary widely across key subgroups. Thus motivated, we develop a framework for incorporating group fairness into the dynamic refugee assignment problem. Equipped with our framework, we propose two bid-price algorithms that maximize overall employment while simultaneously yielding provable group fairness guarantees. Through extensive numerical experiments using real-world data, we show that our algorithms can yield substantial improvements in group fairness compared to state-of-the-art algorithms with only small decreases in global performance.