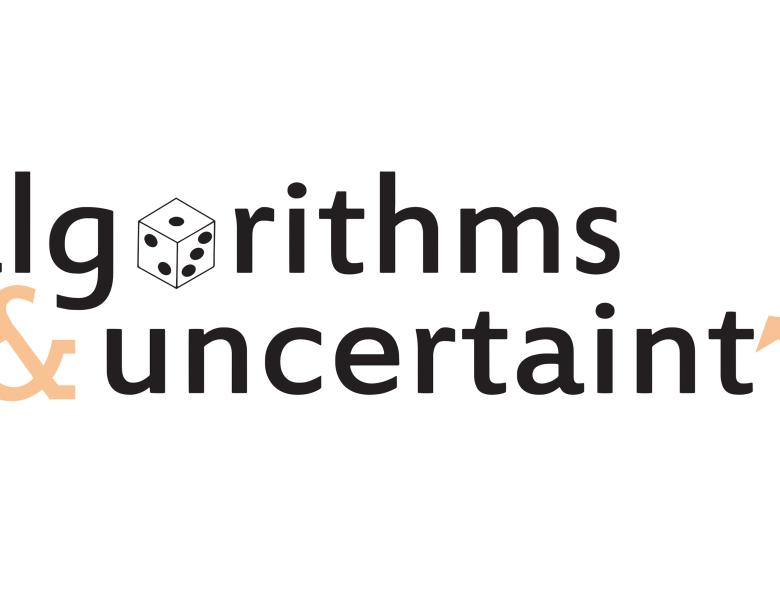
Abstract
We overview several techniques for solving large scale reinforcement learning problems of the type that might commonly arise in advertising and recommendation contexts. We place special emphasis on techniques that exploit the data and models that are used for traditional "myopic" prediction of user behavior (e.g., CTR) to readily construct policies that optimize long-term, cumulative versions of these metrics. We outline challenges and potential solutions that arise in model-free RL in such settings, and derive novel new model-based techniques for the solution of large factored Markov decision processes.We overview several techniques for solving large scale reinforcement learning problems of the type that might commonly arise in advertising and recommendation contexts. We place special emphasis on techniques that exploit the data and models that are used for traditional "myopic" prediction of user behavior (e.g., CTR) to readily construct policies that optimize long-term, cumulative versions of these metrics. We outline challenges and potential solutions that arise in model-free RL in such settings, and derive novel new model-based techniques for the solution of large factored Markov decision processes.