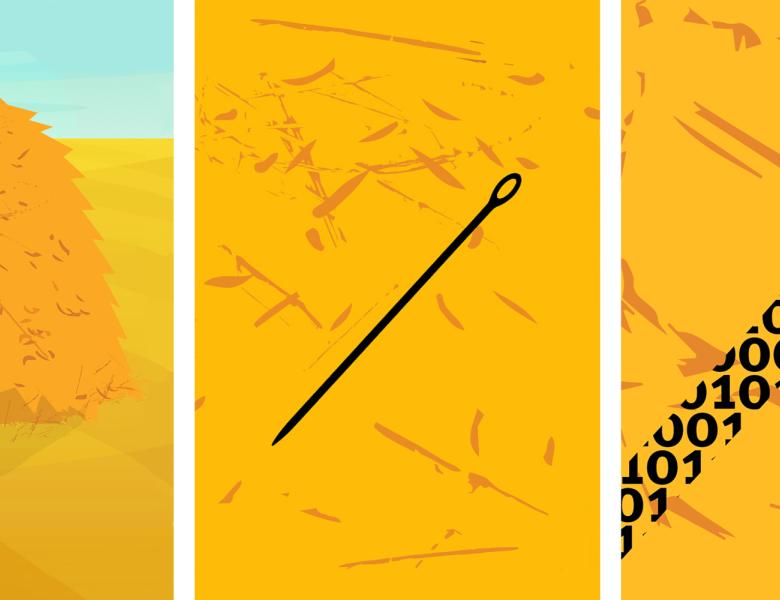
Abstract
Progress in SAT solving has been driven, to a significant extent, by competition - (quasi-)annual solver competitions as well as comparative performance evaluation against state-of-the-art methods from the literature. The same holds for many, if not most, other NP-hard problems in AI, computer science at large, and beyond. In this presentation, I will argue that it is time to rethink the way we assess the state of the art in solving problems such as SAT and the incentives for improving it. I will demonstrate how meta-algorithmic techniques based on sophisticated machine learning and optimisation methods have fundamentally changed not only the state of the art in solving SAT and many other NP-hard problems, but also provide a natural basis for cooperative competition - a new approach for achieving and assessing progress not merely in solving these problems, but also in the way we approach them as a scientific community.