Image
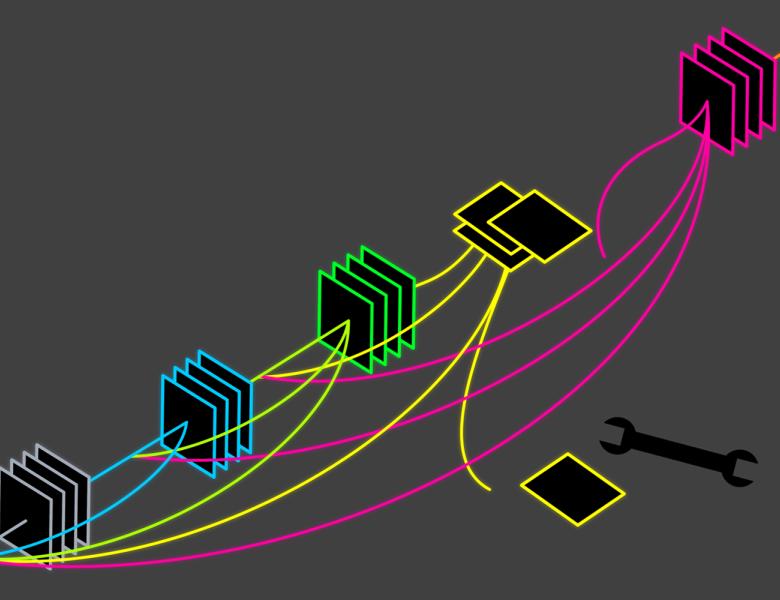
Contrastive learning is an approach to representation learning that uses naturally occurring similar and dissimilar pairs of data points to find useful embeddings of data. We study contrastive learning from the perspective of topic modeling for documents. The main theoretical finding is that, under topic modeling assumptions, contrastive learning recovers a representation of documents that reveals their underlying topic posterior information to linear models. We also empirically demonstrate that linear classifiers with these representations perform well in document classification tasks with very few labeled examples in a semi-supervised setting.
This is joint work with Akshay Krishnamurthy (MSR) and Christopher Tosh (Columbia).