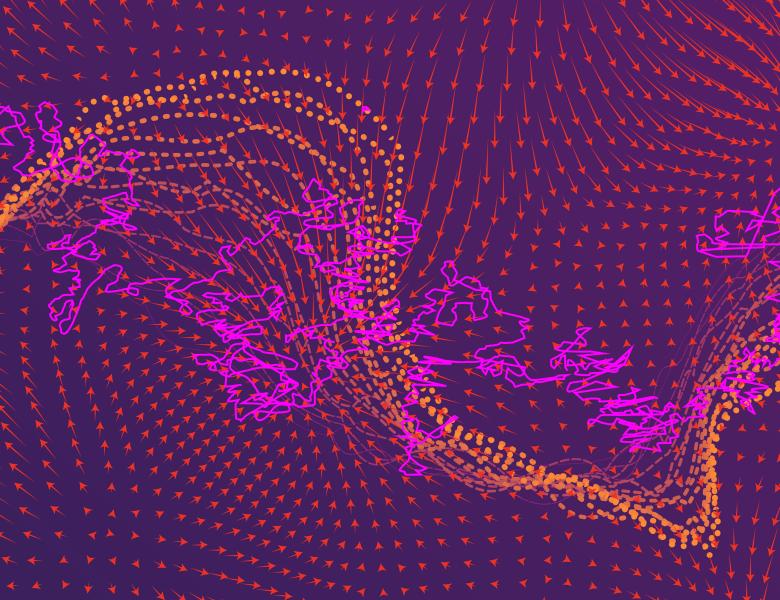
Abstract
The set of minimizers in optimization problems in deep learning is typically either large and high-dimensional or empty. Which minimizer is chosen for practical purposes depends on the choice of optimization algorithm, the initial condition, and hyperparameters of the algorithm. In a continuous time model for stochastic gradient descent, we can analyze the invariant distribution of the algorithm and show that it finds minimizers where the loss landscape is 'flat' in a precise sense. The notion of flatness depends crucially on how the noise intensity at a point scales with the value of the objective function. Under stronger technical conditions, we prove exponential convergence to the invariant distribution.