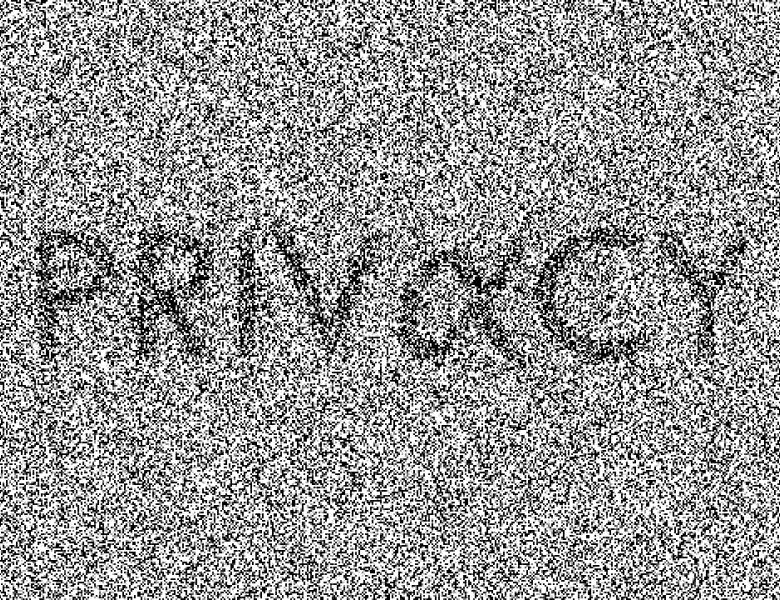
Abstract
Multiple hypothesis testing, a situation when we wish to consider many hypotheses, is a core problem in statistical inference that arises in almost every scientific field. In this setting, controlling the false discovery rate (FDR), which is the expected proportion of type I error, is an important challenge for making meaningful inferences. In this talk, we consider a setting where an ordered (possibly infinite) sequence of hypotheses arrives in a stream, and for each hypothesis we observe a p-value along with a set of features specific to that hypothesis. The decision whether or not to reject the current hypothesis must be made immediately at each timestep, before the next hypothesis is observed. We propose a new class of powerful online testing procedures, where the rejection thresholds are learned sequentially by incorporating contextual information and previous results. Any rule in this class controls online FDR under some standard assumptions. We will also discuss how our proposed procedures would lead to an increase of statistical power over a popular online testing procedure.