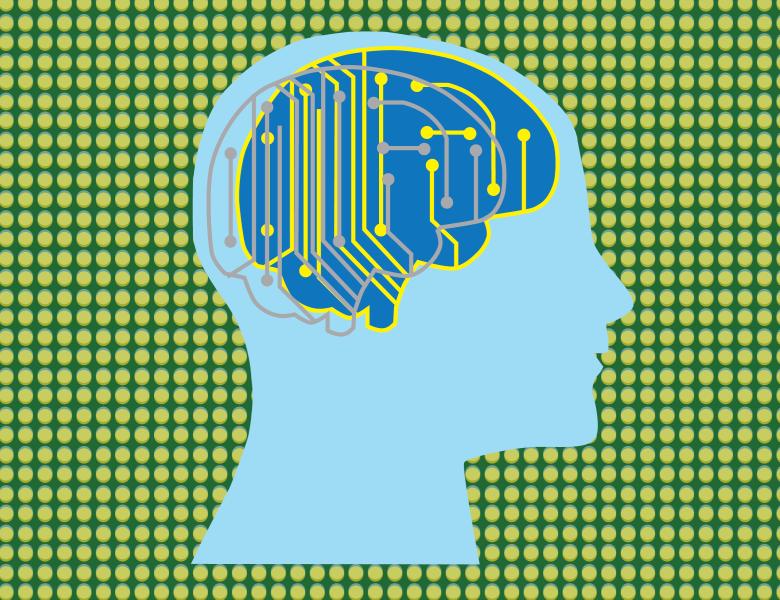
Abstract
We investigate the computational role of the feedback from layer VI of the primary visual cortex (V1) onto LGN relay cells. Our modeling is based on recent experimental results on cat and primate visual cortex that has shed light on the net polarity of feedback as a function of receptive field location of an lgn cell relative to that of a V1 column. These results postulate an excitatory feedback effect when the two receptive field locations largely overlap and an inhibitory feedback when the receptive fields are slightly offset, creating a “Mexican hat”. Our numerical experiments suggest that this feedback scheme results in an increase of information transferred from LGN to V1 when the extent of excitatory and inhibitory regions of the Mexican hat filter are within a range similar to what is observed in the experiments. We further set up an information maximization problem to learn the feedback kernel and observe that the resulting kernel has a Mexican hat shape with similar excitatory and inhibitory widths.