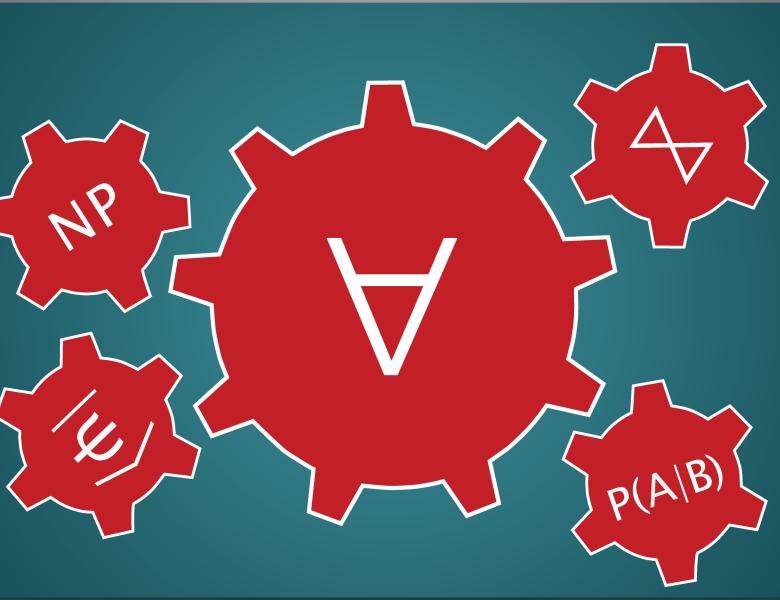
Abstract
Distributional models of natural language use contextual co-occurences to build vectors for words and treat these as semantic representations of the words. These vectors are used for natural language tasks such as entailment, classification, paraphrasing, indexing and so on. Extending the word vectors to phrase and sentence vectors has been the Achilles heel of these models. Building raw vectors for larger language units goes against the distributional hypothesis and suffers from data sparsity. Composition is the way forward. In this talk I will show how category theory can help. I will present categorical models of language based on finite dimensional vector spaces and of Lambek's categorial grammars (residuated monoids, pregroups), joint work with Balkir, Clark, Coecke, Grefenstette, Kartsaklis, and Milajevs. I will also present more recent work, joint with Muskens, on direct vector models of lambda calculus, following the lead of Montague who applied these to natural language. The latter will enable me to relate this work to our preliminary joint work with Abramsky on sheaf theoretic models of language.