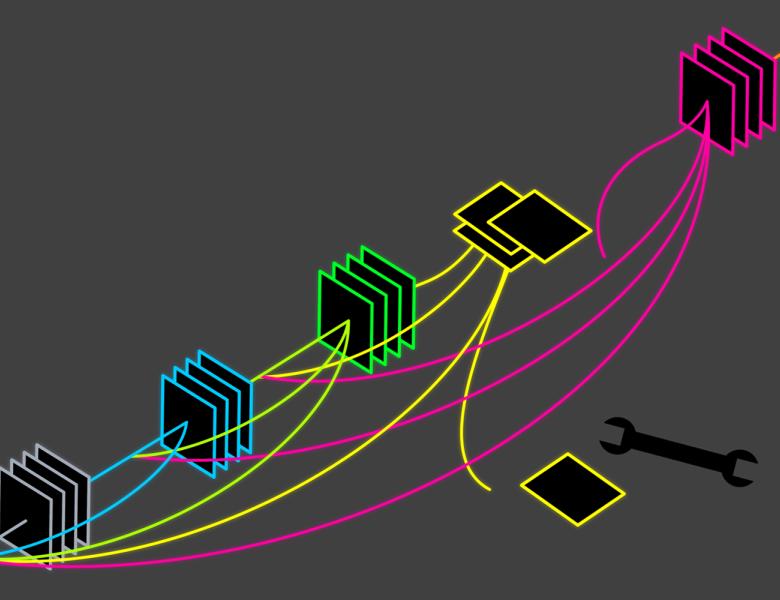
Abstract
We characterize the complexity of finding approximate stationary points (points with gradient norm at most epsilon) of smooth non-convex functions using stochastic first-order methods and beyond. In the well-studied model where algorithms access smooth, potentially non-convex functions through queries to an unbiased stochastic gradient oracle with bounded variance, we prove that any algorithm requires at least $\epsilon^{-4}$ queries to find an $\epsilon$-stationary point. This lower bound is tight, and establishes that stochastic gradient descent is minimax optimal. Moving to higher-order methods, we show that second-order information can improve the complexity to $\epsilon^{-3}$ queries, that this rate is optimal, and---surprisingly---that third-order methods and beyond can offer no further improvement. We then discuss extensions including 1) establishing the optimality of recently proposed variance reduction techniques, and 2) improved algorithms for finding second-order stationary points and matching lower bounds. We conclude with some open problems.