Image
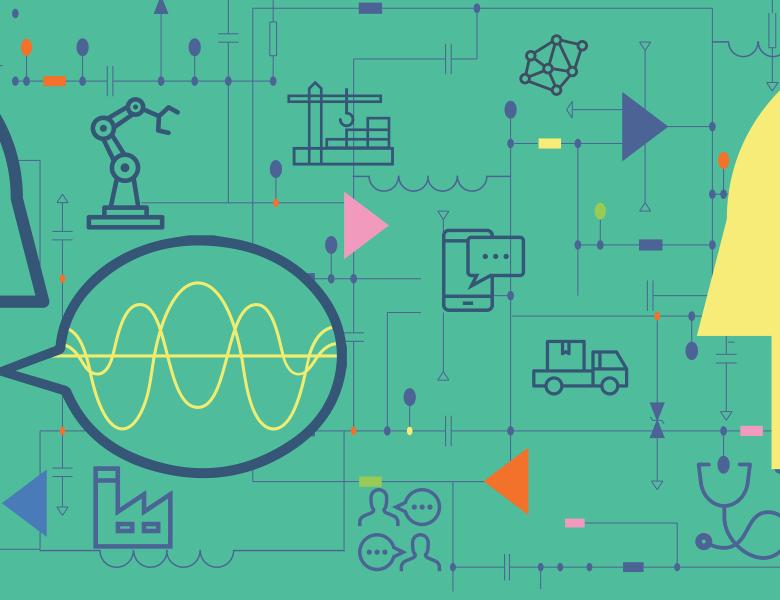
This talk will revolve around two performance criteria that have been studied in the context of episodic reinforcement learning: an old one, Best Policy Identification (BPI) [Fiechter, 1994], and a new one, Reward Free Exploration (RFE) [Jin et al., 2020]. We will see that a variant of the very first BPI algorithm can actually be used for the more challenging reward free exploration problem. This Reward-Free UCRL algorithm, which adaptively explores the MDP and adaptively decides when to stop exploration, requires fewer exploration episodes than state-of-the art algorithms. We will then present alternative algorithms for the BPI objective and discuss the relative complexity of BPI and RFE.