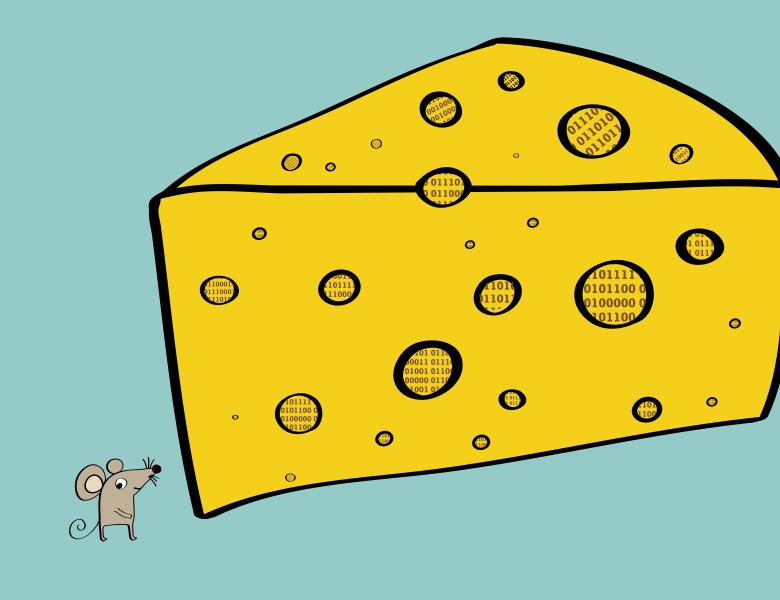
Abstract
Frequency estimation, a.k.a. histograms, a.k.a.(ish) heavy hitters, is a workhorse of data analysis, and as such has been thoroughly studied under differentially privacy. In particular, computing histograms in the local model of privacy (LDP) has been the focus of a fruitful recent line of work, and various algorithms have been proposed, all achieving the order-optimal infinity error while also balancing other considerations such as time- and communication-efficiency. Optimal, as these guarantees are complemented by information-theoretic lower bounds.
However, this is all in the "high-privacy" regime ("small epsilon"). In this talk, I will argue that the (quite common in practice) "low-privacy" regime is not only much less understood, but also that it is surprisingly interesting, both in terms of results and analysis. If time allows, I will also provide some empirical comparison and discussion of how the various existing algorithms perform empirically, beyond their worst-case guarantees.
Joint work with Abigail Gentle.