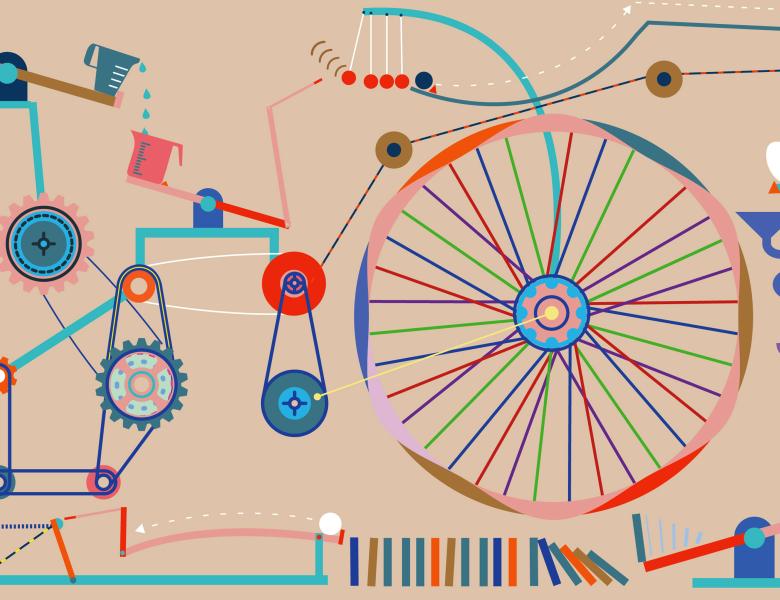
Abstract
Randomized experiments are widely used for investigating causal quantities in a variety of settings, from clinical trials and public policy evaluations to development economics and product development. A standard assumption in designing and analyzing such experiments is that of “no-interference”, which states that a participant’s outcome is only affected by their individual treatment and not by the treatments given to other participants in the experiment. Although standard, this assumption does not hold in many experimental settings, e.g. studies of the effect of vaccines where disease may spread between the participants or cash transfer programs where participants may affect local economies containing other participants. In this talk, I will present a new design-based experimental framework for formulating and investigating causal quantities under complex interference. The framework is expressive in the sense that it allows experimenters to define and investigate new causal estimands based on continuous or discrete treatment assignments under rich and complex interference structures. We present the Riesz Estimator, which is a unified approach to estimation based on insights from functional analysis. Finally, we derive necessary statistical tools (CLT, variance estimators) to construct asymptotically valid confidence intervals for the causal estimand. Joint work with Fredrik Sävje and Yitan Wang.