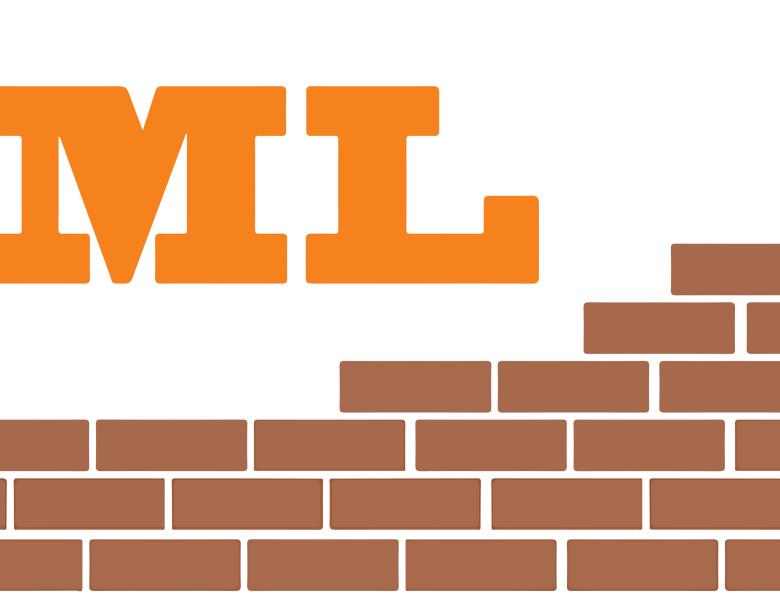
Abstract
Languages synthesize, borrow, and coin new words. This observation is so uncontroversially robust that it is charaterized by empirical laws (Zipf's and Heap's Laws) about the distributions of words and word frequencies rather than by appeal to any particular linguistic theory. However, the first assumption made in most work on word representation learning and language modeling is that a language's vocabulary is fixed, with the (interesting!) long tail of forms replaced with an out-of-vocabulary token, <unk>. In this talk, I discuss the challenges of modeling the statistical facts of language more accurately, rather than the simplifying caracature of linguistic distributions that receives so much attention in the literature. I discuss existing models that relax the closed vocabulary assumption, how these perform, and how they still might be improved.