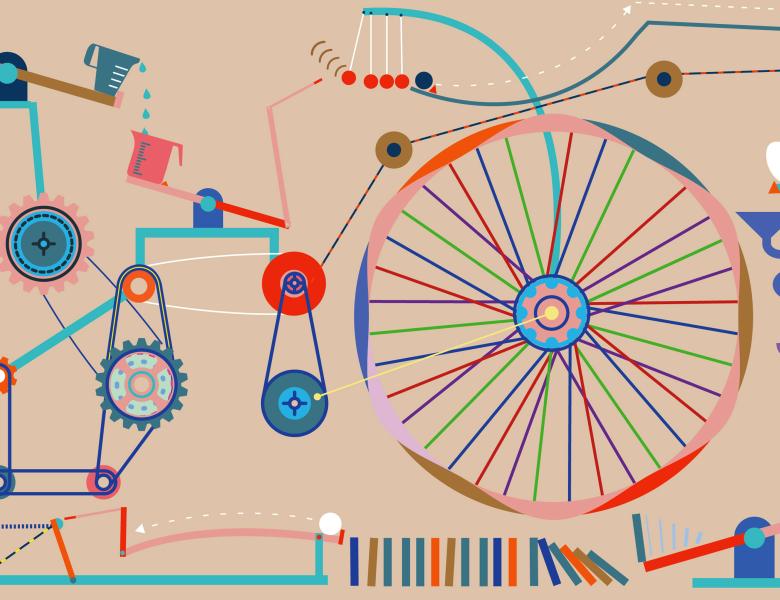
Abstract
In recent years, an interesting duality between experimental and observational approaches to causal inference has become aparent: On the one hand, much insight has been gained into how complex causal relations can be learned better with at least some kind of intervention, even if not fully controlled or partly unknown. This is also related to the concept of exogeneity in economics, where natural experiments are often exploited for causal inference, with methods based for instance on instrumental variables. At the same time, the traditional field of randomized controlled (clinical) trials (RCTs) - with randomization often considered the "gold standard" of causal inference - has come to understand that causal inference reasoning and methods designed for observational data are useful to cope with so called 'intercurrent events', i.e. situations where developments post treatment-assignment 'undo' the randomization. Moreover, it can be valuable to combine an RCT with real-world data to achieve greater generalizability. These developments have highlighted that the formal formulation of the target of inference, the 'estimand', which should reflect the subject matter research question as closely as possible, is an issue in and of itself: 'causal effect' does not equal 'causal effect'! In this talk I will review the above developments and discuss the role of interventions, even if only hypothetical, for the elicitation and formulation of causal research questions and estimands.