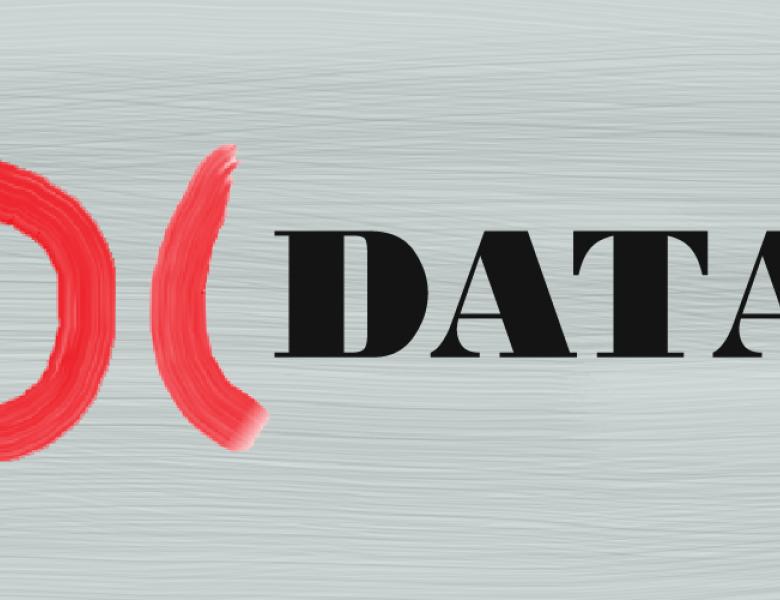
Abstract
Linear Gaussian covariance models are Gaussian models with linear constraints on the covariance matrix. Such models arise in many applications, such as stochastic processes from repeated time series data, Brownian motion tree models used for phylogenetic analyses and network tomography models used for analyzing the connections in the Internet. Maximum likelihood estimation in this model class leads to a non-convex optimization problem that typically has many local maxima. However, I will explain that the log-likelihood function is in fact concave over a large region of the positive definite cone. Using recent results on the asymptotic distribution of extreme eigenvalues of the Wishart distribution I will show that running any hill-climbing method in this region leads to the MLE with high probability.