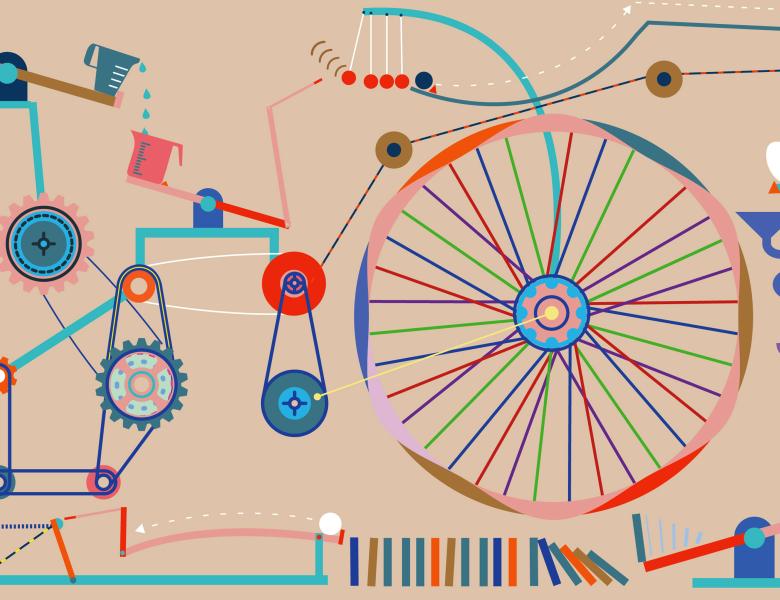
Abstract
Latent variables in directed graphical models can be assumed to have finite cardinality without restricting the marginal model, if all observed variables are discrete. In this talk, we improve on existing non-restrictive cardinality bounds for latent variables by characterizing the dimension of the marginal model to which each latent variable is relevant. We observe that non-restrictive latent variable cardinality bounds depend on whether, and which, counterfactual random variables are being modeled. The improved cardinality bounds facilitate use of the polynomial system characterization to answer causal compatibility and inference questions by reducing the number of unidentified model parameters. Towards the same end, we suggest a 'hybrid' parameterization for latent variable models that yields fewer parameters than existing parameterizations.