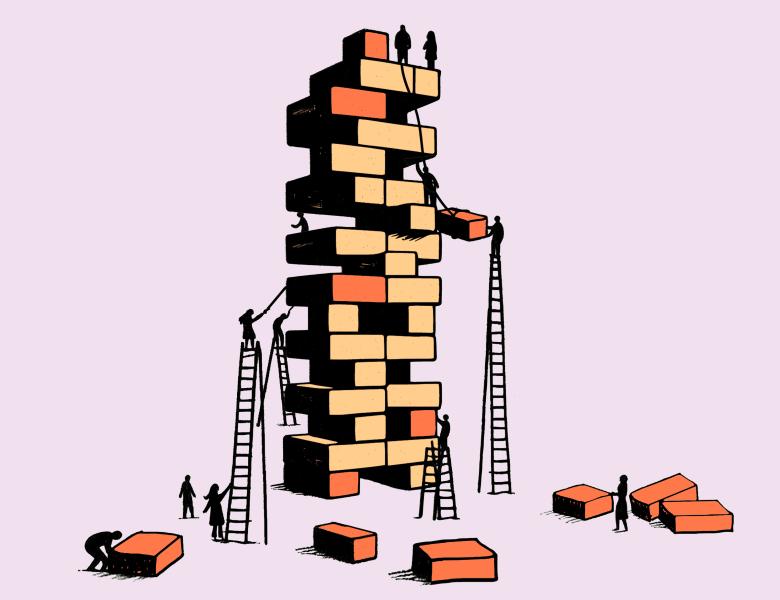
Abstract
One of the major mysteries in science is the towering success of machine learning. In this talk, I will present my work on advancing our theoretical understanding of learning and intelligence through the computational perspective. First, I will talk about the fundamental role of memory in learning, highlighting its importance in continual learning as well as decision making and optimization. Second, I will present an exponential improvement in swap-regret minimization algorithms, which achieves near-optimal computation/communication/iteration complexity for computing a correlated equilibrium, and implies the first polynomial-time algorithm for games with an exponentially large action space (e.g. Bayesian and extensive-form games). Finally, I will talk about learning over evolving data, and conclude the talk with future research directions and my vision for a computational understanding of learning.