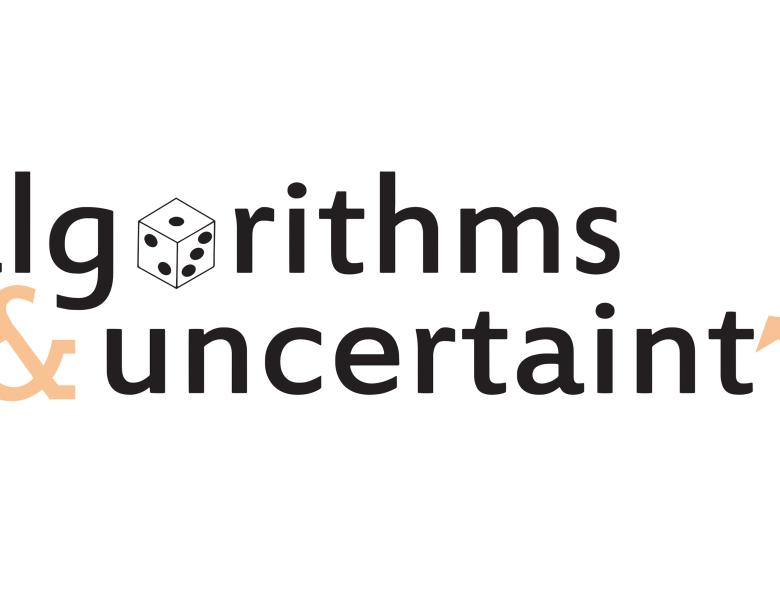
Abstract
An active learner is given a hypothesis class, a large set of unlabeled examples and the ability to interactively query labels of a subset of them; the learner's goal is to learn a hypothesis in the class that fits the data well by making as few label queries as possible. While active learning can yield considerable label savings in the realizable case -- when there is a perfect hypothesis in the class that fits the data -- the savings are not always as substantial when labels provided by the annotator may be noisy or biased. Thus an open question is whether more complex feedback can help active learning in the presence of noise.
In this talk, I will present two separate feedback mechanisms, and talk about when they can help reduce the label complexity of active learning. The first is when labels are obtained from multiple annotators with slightly different labeling patterns; the second is when the annotator can say "I don't know" instead of providing an incorrect label.
This talk is based on joint work with Chicheng Zhang, Songbai Yan and Tara Javidi.