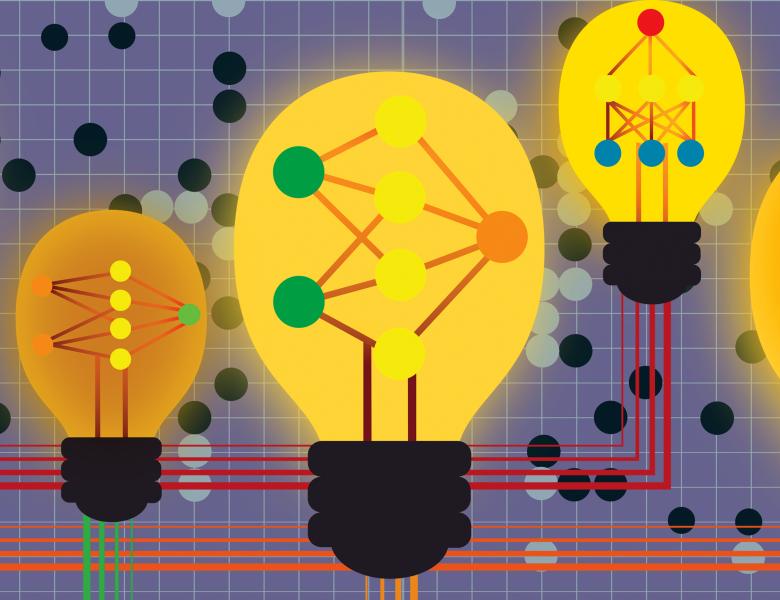
Abstract
Machine learning models suffer from distribution shifts. Over the years, many adversarial approaches have been proposed to improve distributional robustness, especially in the worst-case settings. Representative methods include distributionally robust optimization, adversarial distributional training, and invariant risk minimization. Instead, consistency regularization enforces the learned model to have similar output on augmented data. In this talk, we discuss the connection and limitation of some adversarial approaches compared to consistency regularization. Consistency regularization can provably utilize additional unlabeled samples and propagate a source classifier to target domains while improving the source classifier at the same time. Inspired by our theory, we adapt consistency-based semi-supervised learning methods to domain adaptation settings and gain significant improvements.