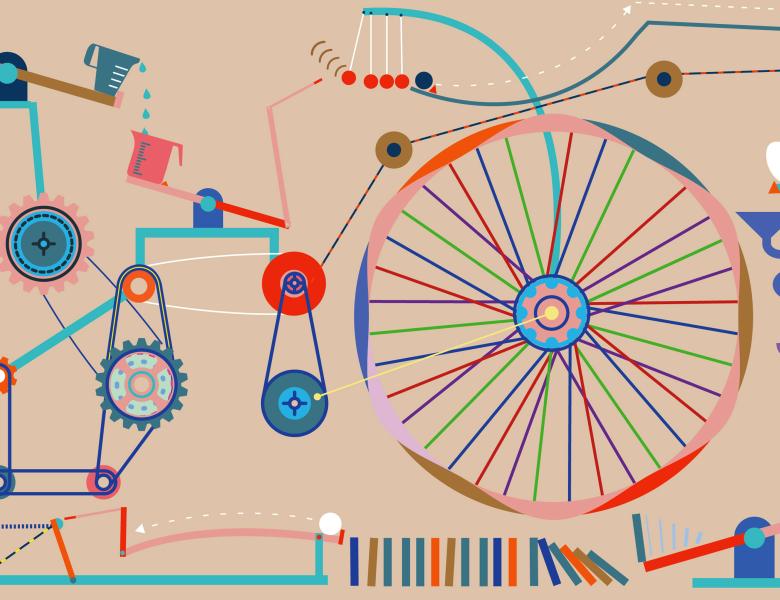
Abstract
Recently, addressing “spatial confounding” has become an important topic in spatial statistics. We trace an influential but ultimately misguided idea about how to control for spatial concounding to a misunderstanding about the connection between random and fixed effects, and prove new results about their equivalence in partially linear models. We then propose a causal inference framework for nonparametric identification of the causal effect of a continuous exposure on an outcome in the presence of spatial confounding. We propose using “double machine learning” (DML) methods, in which flexible models are used to regress both the exposure and outcome variables on confounders to arrive at an estimator with favorable robustness properties and convergence rates. These methods are common in iid settings but underdeveloped for settings with dependence; we prove that this approach results in consistent and asymptotically normal estimators under some forms of spatial dependence. As far as we are aware, this is the first approach to spatial confounding that does not rely on restrictive parametric assumptions (such as linearity, effect homogeneity, or Gaussianity) for both identification and estimation. We demonstrate the advantages of the DML approach analytically and in simulations and apply our methods to a study of the effect of fine particulate matter exposure during pregnancy on birthweight in California.