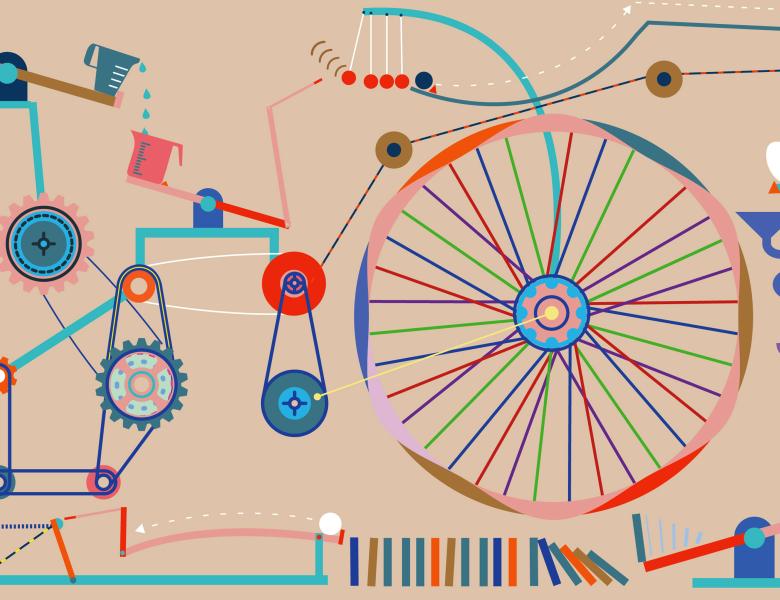
Abstract
One of the main concepts in quantum physics is a density matrix, which is a symmetric positive definite matrix of trace one. Finite probability distributions can be seen as a special case when the density matrix is restricted to be diagonal.
We develop a probability calculus based on these more general distributions that includes definitions of joints, conditionals and formulas that relate these, including analogs of the Theorem of Total Probability and various Bayes rules for the calculation of posterior density matrices. The resulting calculus parallels the familiar ``conventional'' probability calculus and always retains the latter as a special case when all matrices are diagonal.
Whereas the conventional Bayesian methods maintain uncertainty about which model has the highest data likelihood, the generalization maintains uncertainty about which unit direction has the largest variance.
Surprisingly the bounds also generalize: as in the conventional setting we upper bound the negative log likelihood of the data by the negative log likelihood of the MAP estimator.
It is natural to build an "information theory" for density matrices based on the calculus. We conclude with a number of other Bayes rules developed recently and the question of realizability in Physics/Biology.
This is joint work with Dima Kuzmin.