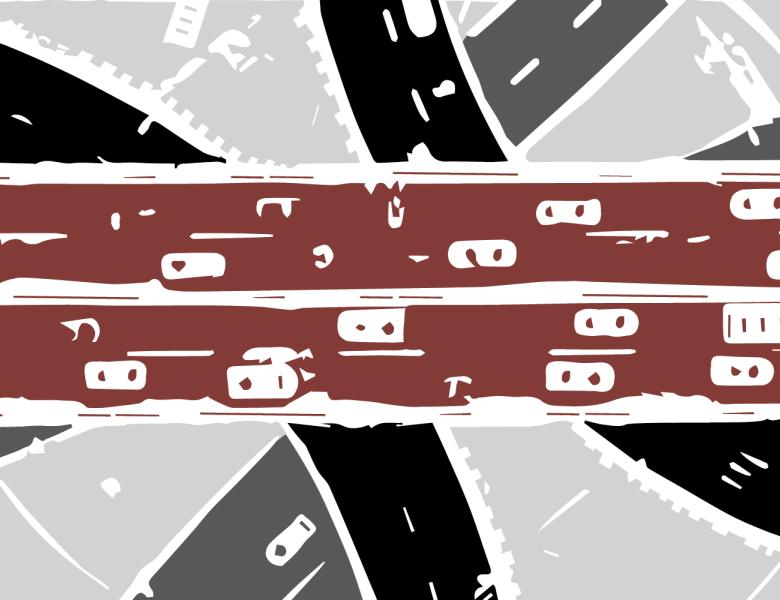
Abstract
In this talk, I will present the latest results on my group’s decade long study of social learning and opinion dynamics. We study the behavioral foundations of non-Bayesian models of learning over social networks and present a taxonomy of conditions for information aggregation in a very general framework. As our main behavioral assumption, we postulate that agents follow social learning rules that satisfy “imperfect recall,” according to which they treat the current beliefs of their neighbors as sufficient statistics for the entire history of their observations. We augment this assumption with various restrictions on how agents process the information provided by their neighbors and obtain representation theorems for the corresponding learning rules (including the popular canonical consensus model of DeGroot). We then obtain general long-run learning results that are not tied to the learning rules’ specific functional forms, thus identifying the fundamental forces that lead to learning, non-learning, and mislearning in social networks. We will also present formal results on complexities of fully Bayesian information aggregation and learning. If time permits, I will also present our new PhD program in Social and Engineering Systems, an interdisciplinary PhD program that combines information and decision theory and social sciences to address complex societal problems.
Joint work with Alireza Tahbaz-Salehi (Kellogg School, Northwestern U), Pooya Molavi (MIT Economics), Amin Rahimian (MIT IDSS).
Paper: http://economics.mit.edu/files/14542 (forthcoming, Econometrica, 2018)