Image
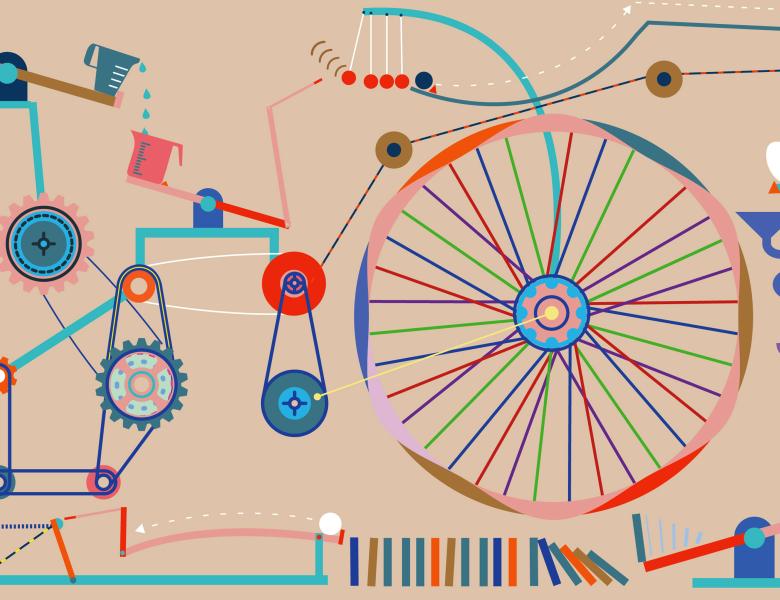
We will review the inflation technique for causal inference. Inflation is a technique for outer-approximating the statistical causal models of marginalized DAGs through a convergent hierarchy of convex relaxations. We will stress how inflation can be adjusted to explore either the (conventional) paradigm of classical latent variables or the more exotic paradigm of nonclassical physical theories such as quantum physics. We will showcase some recent insights obtained though inflation, focusing primarily on results not found in the original publications. Special for this workshop, we will reveal a fundamental connection between do-conditional identifiability and the optimal application of inflation to multilayer DAGs.