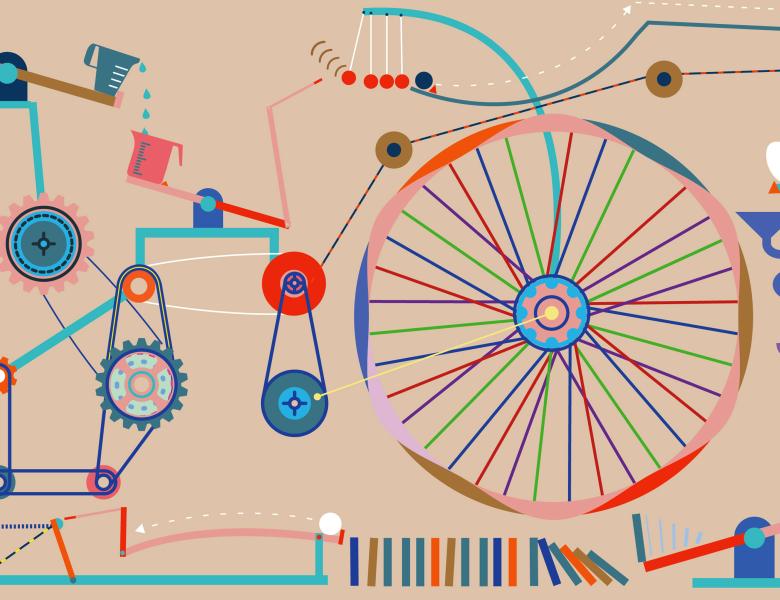
Abstract
We study a constructive algorithm that approximates Gateaux derivatives for statistical functionals by finite-differencing, with a focus on causal inference functionals. We consider the case where probability distributions are not known a priori but also need to be estimated from data. These estimated distributions lead to empirical Gateaux derivatives, and we study the relationships between empirical, numerical, and analytical Gateaux derivatives. Starting with a case study of estimating the mean potential outcome (hence average treatment effect), we establish the exact relationship between finite-differences and the analytical Gateaux derivative in order to derive improved rates of numerical approximation in perturbation and smoothing that preserve the statistical benefits of one-step adjustments, such as rate-double-robustness. We also study more complicated functionals such as dynamic treatment regimes, and optimization-based estimators, with two case studies on the linear-programming formulation for policy optimization in infinite-horizon Markov decision processes and sensitivity analysis in causal inference. The newfound ability to approximate bias adjustments in the presence of arbitrary constraints illustrates the usefulness of constructive approaches for Gateaux derivatives. We find that the statistical structure of the functional (rate-double robustness) can permit less conservative rates of finite-difference approximation, but this depends on the functional. For optimization-based estimators that are marginalizations of linear programs defined on conditional probability distributions, we show that optimization-based arguments based on Danskin's theorem omit additional orthogonalization that is achieved by bias adjustments derived by analyzing the closed-form solution of the optimization program. Finite-differences approximates the statistically sharper estimator.