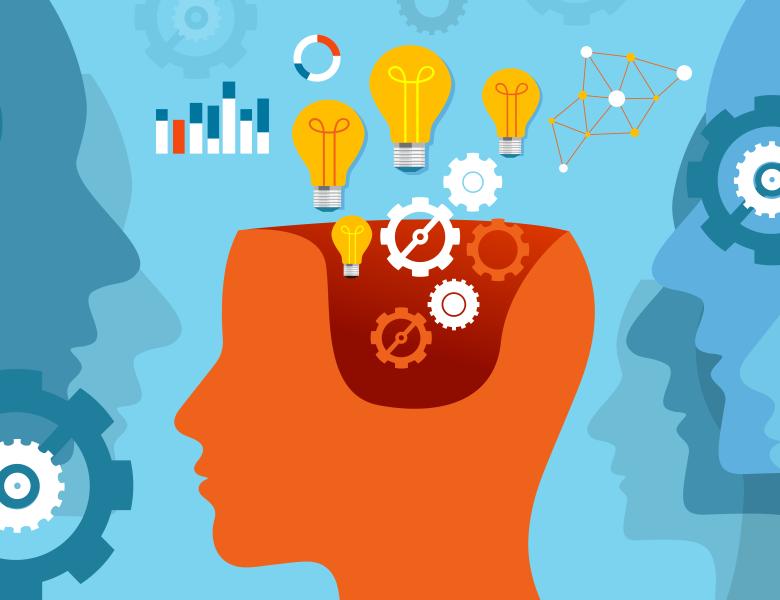
Abstract
Following breakthroughs in using deep learning in such diverse domains as computer vision and natural language processing, a burgeoning line of research leverages deep learning for scientific applications. Partial differential equations (PDEs) are a key primitive in many scientific applications, motivating a rapidly growing area of research in data-driven approaches to solving PDEs. The talk will survey several recent works on understanding PDEs for which neural networks constitute a good choice of a parametric family: in particular, in terms of representational strength, they circumvent "curse of dimensionality" style bounds. We will also show how theoretical insights can be used to elucidate and guide architectural design for neural operators. Based on the works: https://arxiv.org/abs/2103.02138, https://arxiv.org/abs/2210.12101, https://arxiv.org/abs/2312.00234 and https://arxiv.org/abs/2409.02313.