Image
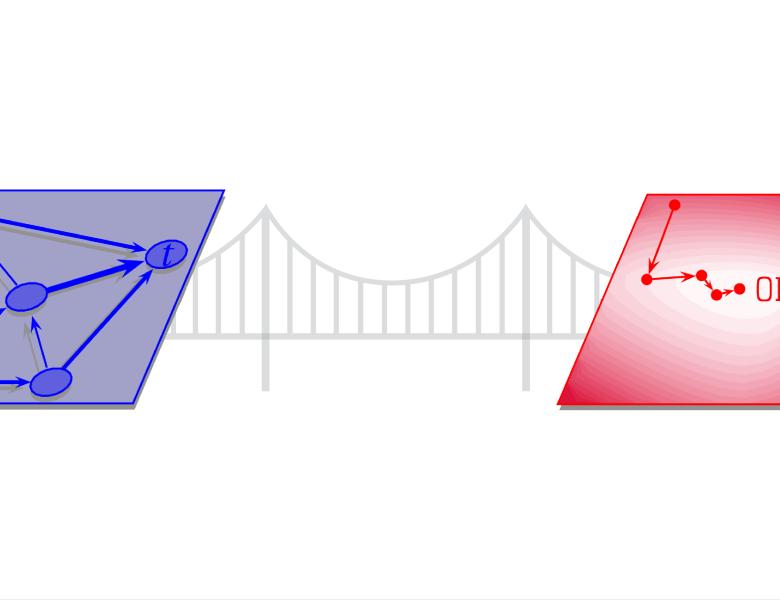
Topic models are extremely useful to extract latent degrees of freedom form large unlabeled datasets. Variational Bayes algorithms are the approach most
commonly used by practitioners to learn topic models. Their appeal lies in the promise of reducing the problem of variational inference to an optimization problem.
I will show that, even within an idealized Bayesian scenario, variational methods display an instability that can lead to misleading results.