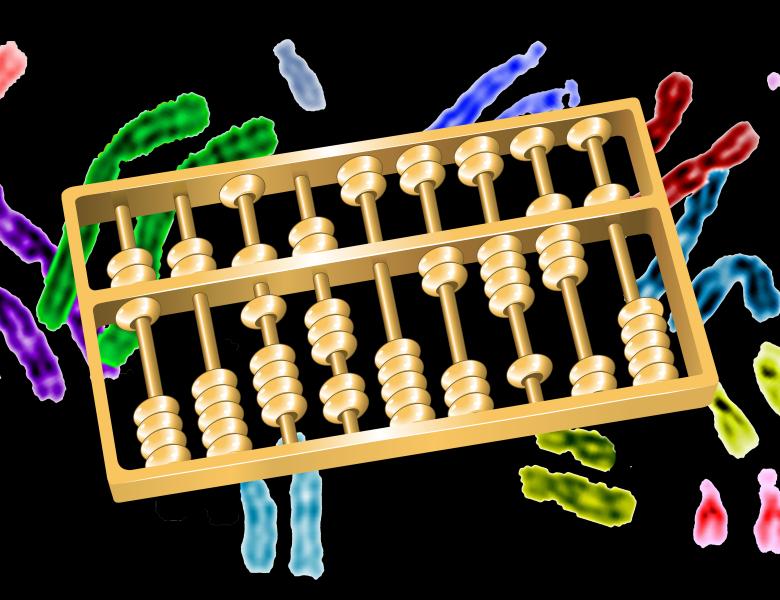
Abstract
DNA sequencing technologies now allow the collection of somatic mutations in a large number of patients from the same cancer type. One of the main goals in the analysis of such datasets is the identification of driver mutations associated with cancer, distinguishing them from random, passenger mutations. This goal is hindered by the extensive genetic heterogeneity in cancer, with different genes mutated in different patients. This heterogeneity is due to the fact that genes and mutations act in the context of networks, with groups of interacting genes (pathways) that perform different cellular functions, and each function can be altered by mutating any of the genes in the pathway.
I will discuss two problems that arise in the analysis of cancer somatic mutations in network contexts. The first one is the problem of finding connected subnetworks of a large gene-gene interaction network that are mutated in a large number of patients, that we prove is NP-hard. I will present a polynomial time approximation algorithm that we designed to identify subnetworks with provable guarantees on their quality. I will also present a recent ILP formulation that identifies solutions of better quality compared to the approximation algorithm and is much faster on data from large cancer studies.
The second problem is the problem of identifying subnetworks of a large gene-gene interaction network that have somatic mutations associated with survival from genome-wide mutation data of a large cohort of cancer patients. We formally define the associated computational problem by using a score for sub networks based on the test statistic of the log-rank test, a widely used statistical test for comparing the survival of two given populations. We show that the computational problem is NP-hard in general and that it remains NP-hard even when restricted to graphs with at least one node of large degree, the case of interest for gene-gene interaction networks. I will present Network of Mutations Associated with Survival (NoMAS), a novel color-coding based algorithm that we have designed to find subnetworks of a large interaction network whose mutations are associated with survival time. I will present results of the application of NoMAS on large cancer datasets.