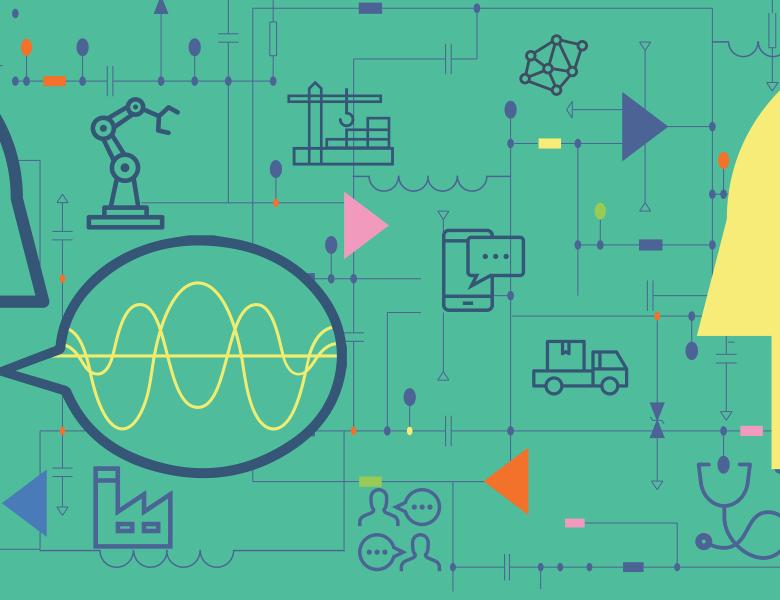
Abstract
Model-free reinforcement learning algorithms combined with value function approximation have recently achieved impressive performance in a variety of application domains. However, theoretical understanding of such algorithms is limited, and existing results are largely focused on episodic or discounted Markov decision processes (MDPs). In this talk, will present adaptive approximate policy iteration (AAPI), a learning scheme which enjoys a O(T^{2/3}) regret bound in undiscounted ergodic MDPs with function approximation. The algorithm and analysis rely on online learning techniques, where action-value functions are treated as losses. The main technical novelty compared to existing work is the use of a data-dependent adaptive learning rate, coupled with a so-called optimistic prediction of upcoming losses.