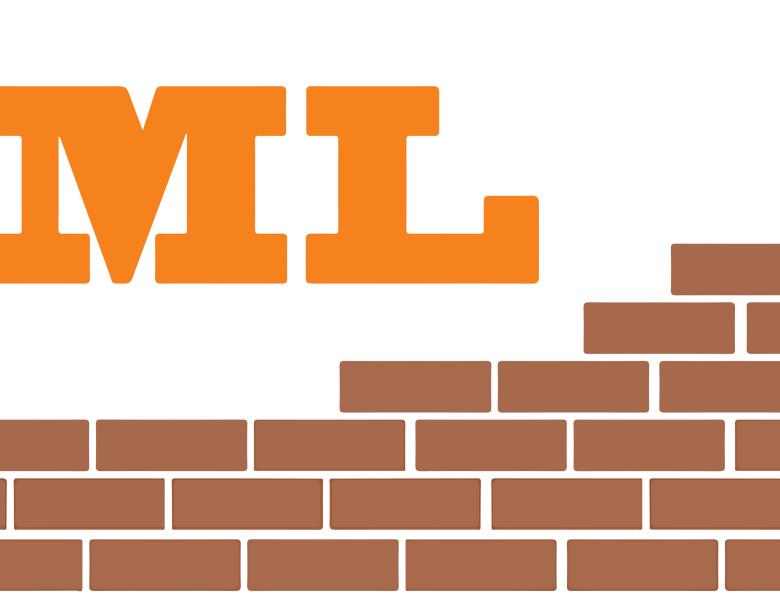
Abstract
Classical experimental design problems in statistics tend to have combinatorial solutions. We consider computationally tractable methods for the experimental design problem, where k out of n design points of dimension p are selected so that certain optimality criteria are approximately satisfied. We prove a constant approximation ratio under a very weak condition that k > 2p, and a (1 + eps) relative approximation ratio under
slightly stronger conditions in which k is still a linear function of p. Numerical results on both synthetic and real-world design problems verify the practical effectiveness of the proposed algorithm.