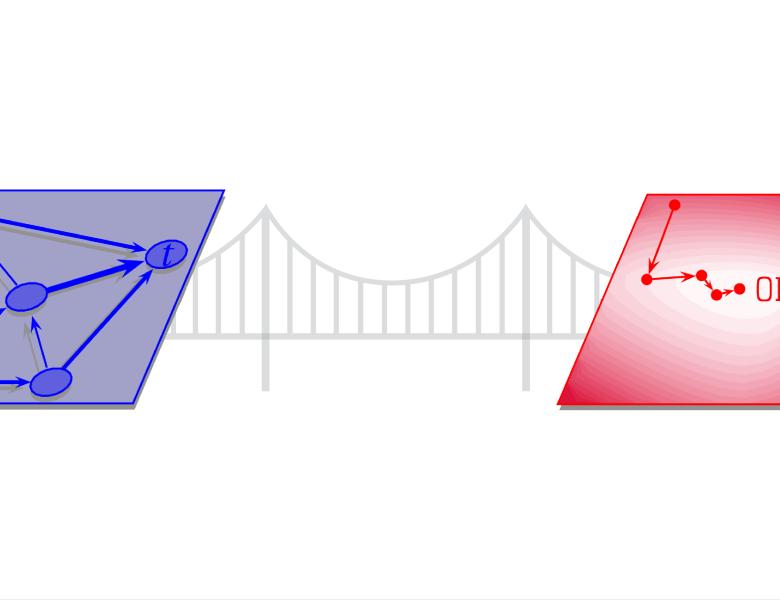
With ubiquitous automated decision-making comes great responsibility (or does it?). Modern algorithms shape and affect almost all aspects of modern life -- search, social media, news, e-commerce, finance, urban transportation, and criminal justice, to name a few. It is becoming urgent that we understand (and mitigate) the unintended consequences of automated decisions to avoid discrimination among the users, as well as ensure due process and transparency in decision-making. For instance, (i) delivery of certain services has been made available at a higher cost based on the location of the customers thus inadvertently discriminating against minority neighborhoods, (ii) algorithms like COMPAS used in the criminal justice system have been shown to incorrectly classify black defendants as high risk with much higher likelihood than white defendants, and (iii) experiments have shown that women are much less likely to be shown higher paying jobs compared to men. These are just some examples in which automated decisions have had adverse effects on various members of the population.
In this mini-symposium, we will discuss what fairness means for supervised learning algorithms, delve deeper into COMPAS and bias in the prediction of the risk of recidivism, and debate whether bias is a social or a mathematical concept.