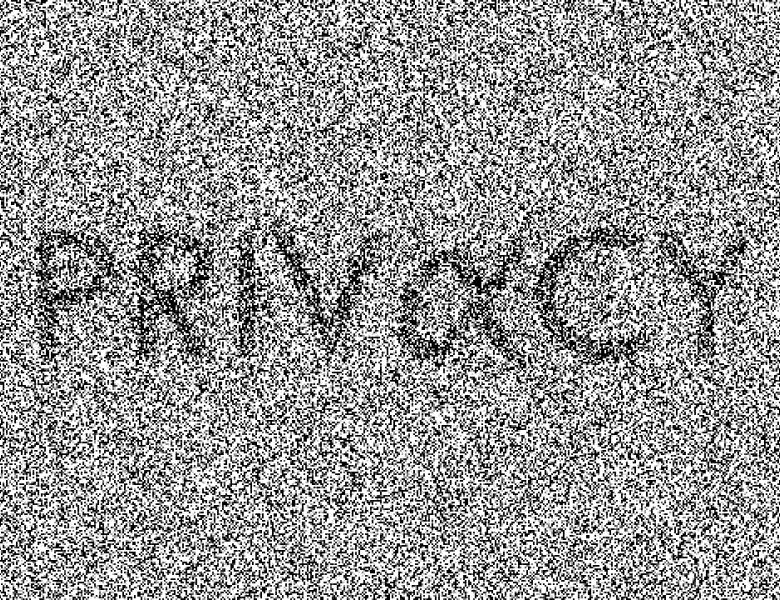
Modern data analysis relies on solutions to a diverse set of inference and prediction problems. Imposing differential privacy or other formal privacy constraints can have a substantial impact on the computational and statistical efficiency with which these problems can be solved. The first theme that this workshop will explore is the frontiers and challenges of solving the common data analysis tasks subject to formal privacy constraints, with a focus on algorithmic and lower bound techniques that illuminate the computational and statistical costs of private data analysis. The second theme of the workshop is the connections between differential privacy viewed as a type of stability and the notions of algorithmic stability studied in learning theory and statistics. This connection provides a promising direction for dealing with the risk of overfitting and false discovery that arise in the challenging adaptive data analysis setting. The workshop will explore these additional connections between the techniques and tools used in private data analysis and techniques aimed at ensuring validity and robustness of statistical data analyses.
Jayadev Acharya (Cornell University), Dimitris Achlioptas (UC Santa Cruz), Brendan Avent (University of Southern California), Jordan Awan (Pennsylvania State University), Borja Balle (Amazon), Andres Felipe Barrientos (Duke University), Raef Bassily (Ohio State University), Johes Bater (Northwestern University), Fattaneh Bayatbabolghani (University of Toronto), Amos Beimel (Ben Gurion University), Abhishek Bhowmick (Apple), Mark Bun (Princeton University), Alexandra Calhoun (Harvard University), Albert Cheu (Northeastern University), Aloni Cohen (Massachusetts Institute of Technology), Rachel Cummings (Georgia Institute of Technology), Frances Ding (Harvard University), Fei Dong (Apple), John Duchi (Stanford University), Cynthia Dwork (Harvard University & Microsoft Research), Alex Edmonds (University of Toronto), Gian Pietro Farina (University at Buffalo, SUNY), Will Fithian (UC Berkeley), Julien Freudiger (Apple), Marco Gaboardi (University at Buffalo, SUNY), Abhradeep Guha Thakurta (UC Santa Cruz), Moritz Hardt (UC Berkeley), Chris Jay Hoofnagle (UC Berkeley), Stanley Hung (Apple), Omid Javidbakht (Apple), Konstantin Kakaes (MIT Technology Review), Gautam Kamath (Massachusetts Institute of Technology), Guarav Kapoor (Apple), Dick Karp (UC Berkeley), Vishesh Karwa (Ohio State University), Shiva Kasiviswanathan (Samsung Research America), Aaron Koolyk (Hebrew University), Aleksandra Korolova (University of Southern California), Frauke Kreuter (University of Maryland), Janardhan Kulkarni (Microsoft Research Redmond), Paul Laskowski (UC Berkeley), Rachel Lawrence (UC Berkeley), Philip LeClerc (US Census Bureau), Katrina Ligett (Hebrew University of Jerusalem), Mike Luby (ICSI), Yuliia Lut (Georgia Institute of Technology), Audra McMillan (Boston University), Carlo Molinari (Pennsylvania State University), Shay Moran (Princeton University), Deirdre Mulligan (UC Berkeley), Anupama Nandi (Ohio State University), Marcel Neunhoeffer (University of Mannheim), Sasho Nikolov (University of Toronto), Helen Nissenbaum (Cornell Tech), Kobbi Nissim (Georgetown University), Elizabeth O’Neill (Cornell Tech), Mijung Park (Max Planck Institute for Intelligent Systems, Tuebingen), Srinivasan Raghuraman (Massachusetts Institute of Technology), Sofya Raskhodnikova (Boston University), Matthew Reimherr (Pennsylvania State University), Omer Reingold (Stanford University), Alessandro Rinaldo (Carnegie Mellon University), Ryan Rogers (Apple), Aaron Roth (University of Pennsylvania), Benjamin Rubinstein (University of Melbourne), Lalitha Sankar (Arizona State University), Adam Sealfon (Massachusetts Institute of Technology), Or Sheffet (University of Alberta), Moshe Shenfeld (Hebrew University), Natalie Shlomo (University of Manchester), Vikrant Singhal (Northeastern University), Shuang Song (UCSD), Thomas Steinke (IBM Almaden), Ramesh Krishnan Pallavoor Suresh (Boston University), Kunal Talwar (Google), Om Thakkar (Boston University), Di Wang (University at Buffalo, SUNY), Yu-Xiang Wang (UC Santa Barbara), Steven Wu (University of Minnesota Twin Cities), Jared Zerbe (Apple), Juba Ziani (Caltech)