This Semester at the Simons Institute, Spring 2019
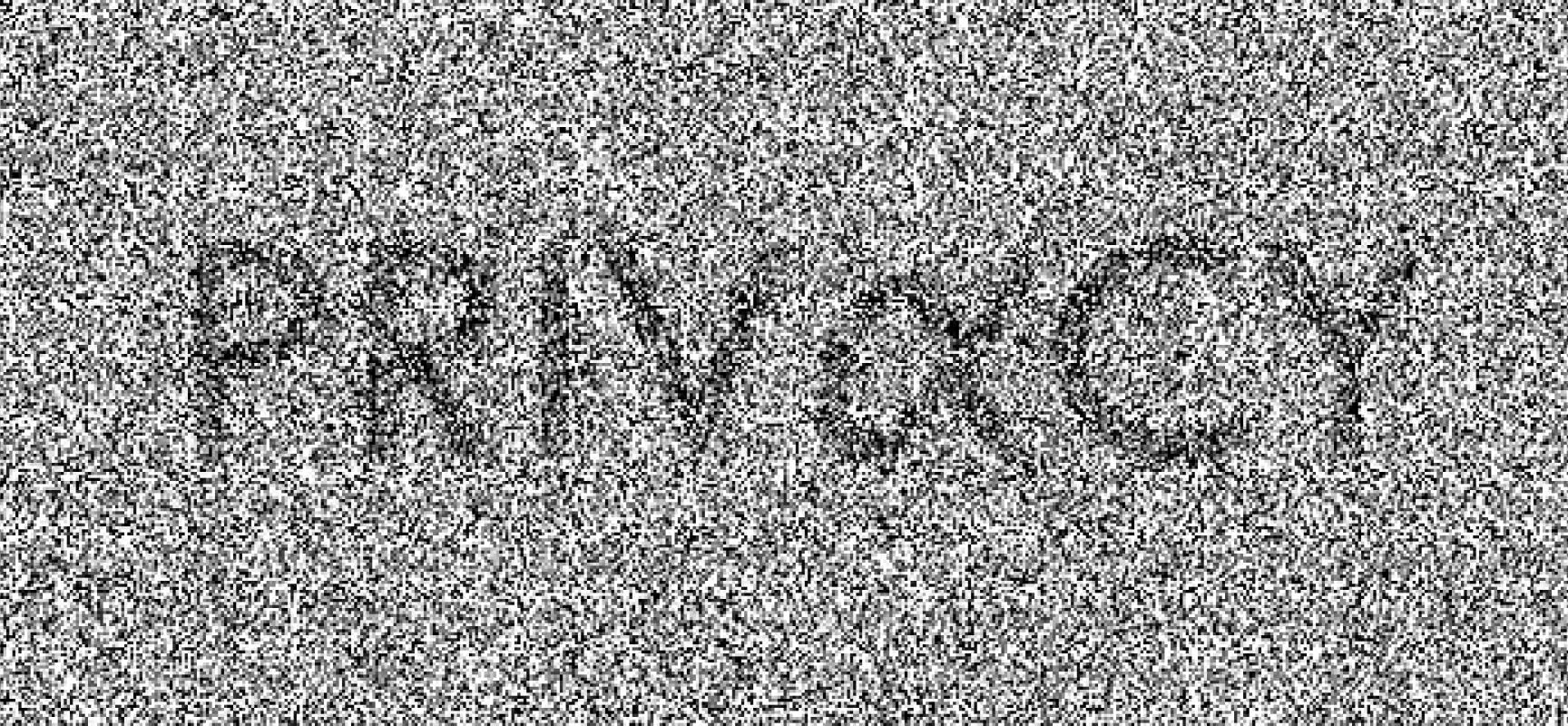
by Luca Trevisan (Simons Institute, UC Berkeley)
This semester, the Simons Institute is hosting a program on data privacy and a program on polynomial methods in combinatorics.
The program on Data Privacy: Foundations and Applications is organized by Vitaly Feldman, Katrina Ligett, Kobbi Nissim, Aleksandra Slavković and Adam Smith. This program has brought to Berkeley a diverse set of scholars interested in algorithmic, statistical, legal and social issues in data privacy. The program is taking place at a timely moment, a year after the implementation of GDPR in Europe, a year before the 2020 census in the United States, and at a time of increasing concern about the treatment of data at several large technology companies.
The first workshop of the program was on applications of data privacy, considering the needs of government agencies, the challenges of industry implementations, and best practices and possible standards. The second workshop focused on how to introduce privacy considerations in data science applications, and the third workshop will look ahead at new approaches to quantitative data privacy that go beyond the paradigm of differential privacy.
The program on Geometry of Polynomials is organized by Leonid Gurvits, Shayan Oveis Gharan, Pablo Parrilo, Alistair Sinclair and Nikhil Srivastava. Unlike many other Simons Institute programs, which target an application domain and gather scholars who study that application domain from several points of view, the Geometry of Polynomials program is characterized by a common mathematical core that has applications in several domains, such as analysis of algorithms, combinatorial constructions, and counting problems. The common mathematical core is the study of the roots of real-rooted polynomials, treating them as geometric objects, as done in algebraic geometry, but with techniques that borrow from analysis.
In the past decade, several important advances — such as the construction of Ramanujan graphs of every degree, approximation algorithms for the asymmetric TSP, and results on the complexity of several counting problems related to statistical physics — have relied in one way or another on examining the zeros of certain real-rooted multivariate polynomials derived from combinatorial objects, and the program will explore new application domains for these new ideas (including hyperbolic programming, a generalization of semidefinite programming) as well as a new synthesis of existing ideas.
The first workshop explored how such methods can go beyond the classic probabilistic method to prove the existence of combinatorial objects and to round solutions of convex relaxations. The second workshop looked at deterministic algorithms for counting problems, with significant interactions with statistical physics. The third workshop will look at hyperbolic polynomials and at other convex optimization techniques that generalize semidefinite programming.
During the coming summer, the Institute will host a full program on Foundations of Deep Learning and two smaller summer clusters, on Fairness and on Error-Correcting Codes and High-Dimensional Expansion.
The program on deep learning will focus on four main topics: how deep neural networks fit data, the energy landscape of loss minimization, how models trained to fit data generalize, how robust they are to adversarially selected outliers, and how to train neural models to generate distributions. The program will start with a boot camp and will feature two additional workshops.
The summer cluster on algorithmic fairness continues, on a larger scale, the activities of a cluster on fairness from summer 2018, and it will feature two workshops. The summer cluster on high-dimensional expanders explores a fascinating new topic in pure mathematics, and it will look at applications to error-correcting codes. It will start with a boot camp.