Program Retrospective: Real Analysis in Computer Science
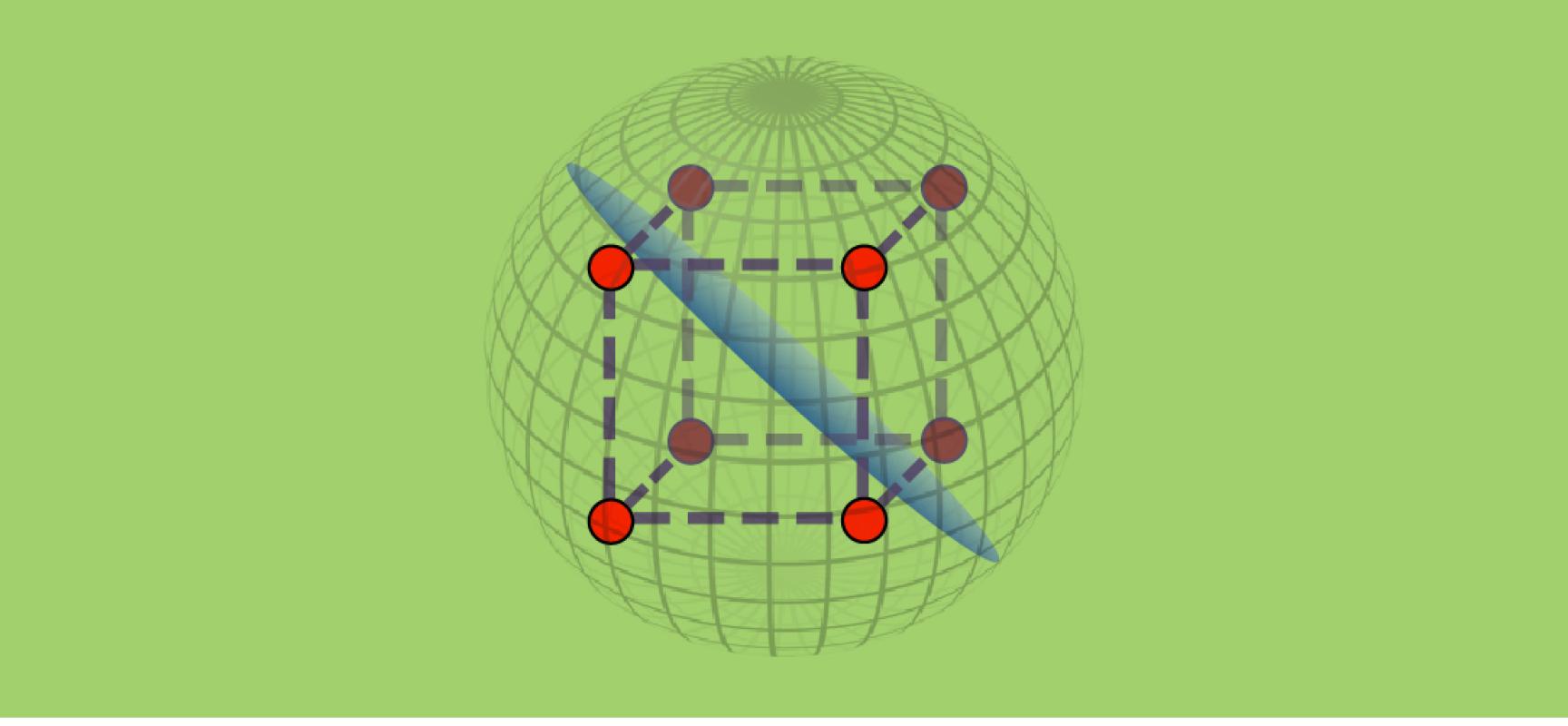
By Prahladh Harsha
The last few decades in theoretical computer science have witnessed the use of analytical tools to study the properties of several types of discrete objects. Results in discrete analysis play an important role in areas as diverse as hardness of approximation, computational learning, computational social choice and communication complexity. A seminal example of this phenomenon is the classical Kahn-Kalai-Linial paper, which demonstrates the use of hypercontractive inequalities in the study of Boolean functions. Another example is the use of Fourier analysis in the study of optimal inapproximability results, as shown by Håstad. A more recent example is the “majority is stablest” theorem of Mossel, O'Donnell and Oleszkiewicz, which was studied in the context of understanding the approximability of the MAXCUT problem. The Simons Institute program on "Real Analysis in Computer Science" brought together many experts from computer science, mathematics, probability and statistics to study these and related phenomena in mathematical analysis, and their applications to theoretical computer science. This program was one of the first semester-long programs (along with the parallel program on Big Data), which were organized in the new Institute during Fall 2013.
The program was kickstarted by a workshop on the applications of real analysis in testing, learning and inapproximability. This first week of the program was spent identifying challenges from learning, computational complexity, and hardness of approximation that could be explicitly expressed in discrete analytic terms. The program participants consisted of a diverse set of researchers, not only from various fields of computer science and mathematics but also from various stages in their careers – senior graduate students, postdoctoral fellows, and more senior researchers. To acquaint this diverse set of participants with the key themes of the program, as well as to set a common vocabulary, the first workshop was followed by a “boot camp” in the second week of September. The boot camp consisted of three mini courses: Inapproximability of Constraint Satisfaction Problems (by Johan Håstad), Analytic Methods for Supervised Learning (by Adam Klivans) and Introduction to Analysis on the Discrete Cube (by Krzysztof Oleszkiewicz). This was then followed by a second workshop on Functional Inequalities in Discrete Spaces with Applications in the first week of October. The focus of this workshop was spectral inequalities, logarithmic Sobolev inequalities and hyper-contractive inequalities in discrete settings and their applications. The Real Analysis Day was organized immediately following the FOCS conference, and featured more detailed versions of four of the topical results from the conference. The final workshop (held in the first week of December) was on Neo-Classical Methods in Discrete Analysis. The topics covered in this workshop were non-commutative Fourier analysis, representation theory techniques, and techniques from additive combinatorics, with emphasis on emerging topics that are yet to be explored in detail. Nati Linial and Johan Håstad gave Open Lectures on “How (and Why) to 'Read' Big Graphs,” and “How Hard Is It To Find A Good Solution?” respectively. These workshops and lectures were accompanied by a graduate course on Analysis of Boolean Functions offered by Gil Kalai, and a weekly real analysis seminar attended by the program participants.
In summary, the Real Analysis program at the Simons Institute provided an exciting forum for the exchange of ideas and intensive collaboration among mathematicians and CS theorists, ranging from senior leaders of the field to the large and vibrant community of postdoctoral fellows.
Related Articles:
Letter from the Director
The Renovation of Calvin Lab: A Photo Essay
Current and Upcoming Programs
Program Retrospective: Theoretical Foundations of Big Data Analysis
Life at the Simons Institute: A Fellow's Perspective
Official Opening of the Simons Institute