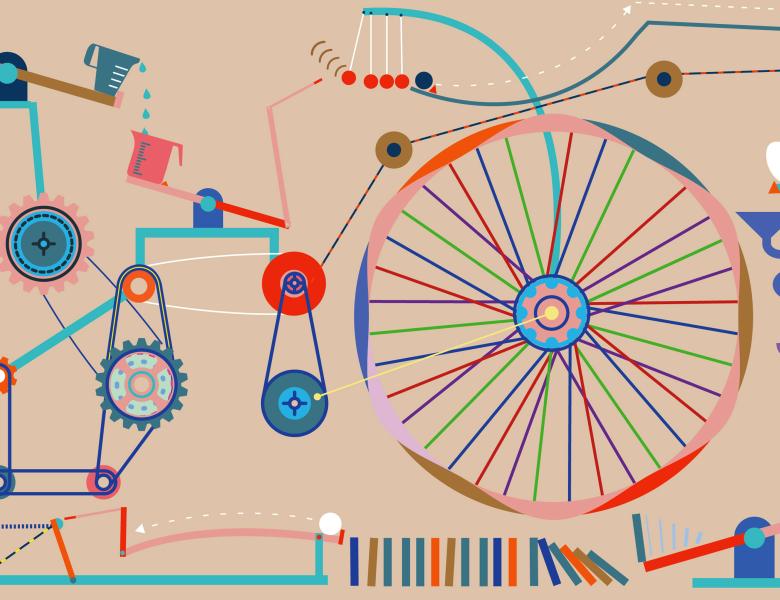
Abstract
Classical approaches to experimental design rely on critical independence assumptions that are violated when the outcome of an individual i may be affected by the treatment of another individual j, referred to as network interference. This interference introduces computational and statistical challenges to causal inference. We present a new hierarchy of models and estimators that enable statistically efficient and computationally simple solutions under nonparametric polynomial models, with theoretical guarantees even in settings without graph knowledge, or under model misspecification.