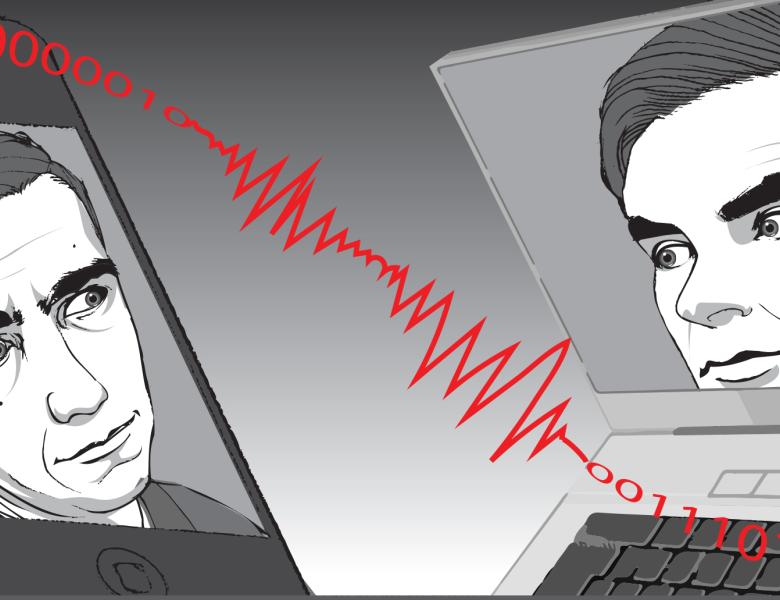
Abstract
Estimation of low-rank matrices is of significant interest in a range of contemporary applications. In this talk we introduce a rank-one projection model for low-rank matrix recovery and propose a constrained nuclear norm minimization method for stable recovery of low-rank matrices in the noisy case. The procedure is adaptive to the rank and robust against small perturbations. The proposed estimator is shown to be rate-optimal under certain conditions. Applications to phase retrieval and estimation of spiked covariance matrices will also be discussed.