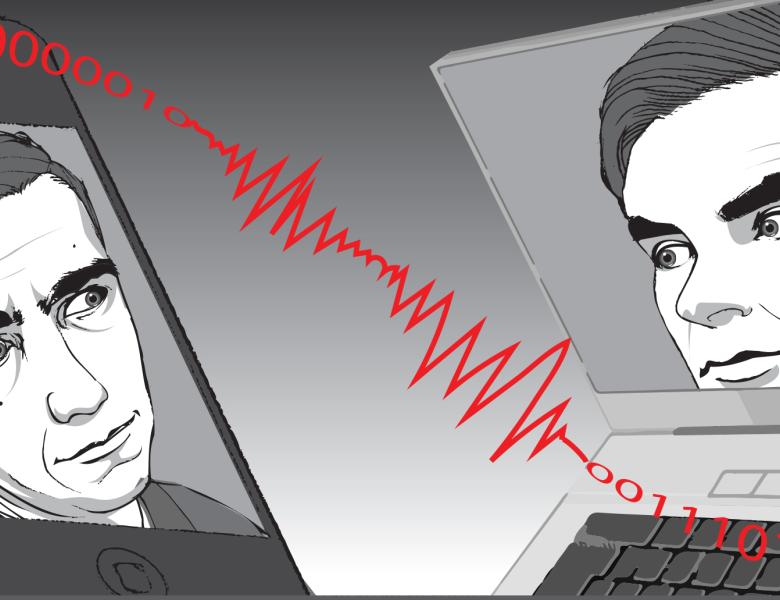
Abstract
Increasingly, optimization problems in machine learning, especially those arising from high-dimensional statistical estimation tasks, involve a large number of variables. As regards the statistical estimation tasks themselves, methods developed over the past decade have been shown to have statistical or sample complexity that depends only weakly on the number of parameters, when there is some structure to the problem, such as sparsity. A central question is whether similar advances can be made in their computational complexity as well. In this talk, we propose strategies that indicate that such advances can indeed be made. In particular, we investigate the greedy coordinate descent algorithm, and note that performing the greedy step efficiently weakens the costly dependence on the problem size provided the solution is sparse. We then propose a suite of methods that perform these greedy steps efficiently by a reduction to nearest neighbor search. One specialization of our algorithm combines greedy coordinate descent with locality sensitive hashing, using which we are not only able to significantly speed up the vanilla greedy method, but also outperform cyclic descent when the problem size becomes large.
Joint work with Inderjit Dhillon and Ambuj Tewari.