Image
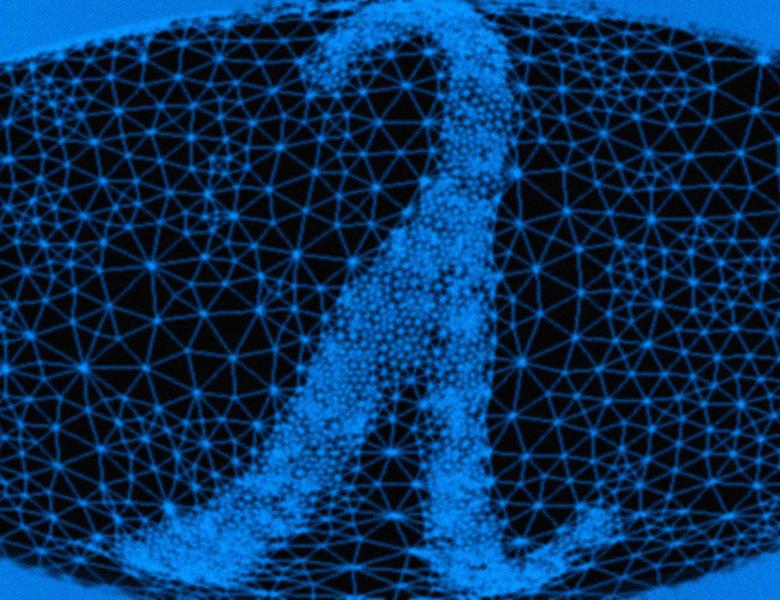
Low rank tensor decompositions are a powerful tool for learning generative models, and uniqueness results give them a significant advantage over matrix decomposition methods. In this talk, I will describe two recent results:
(a) a robust version of a classical uniqueness theorem of Kruskal for tensor decompositions which immediately implies polynomial identifiability in many settings.
(b) a smoothed analysis framework for analyzing algorithms for tensor decomposition which models realistic instances of learning problems and allows us to overcome many of the usual limitations of using tensor methods.
Based on joint works with Aditya Bhaskara, Ankur Moitra and Aravindan Vijayaraghavan.